Behind the Scenes: Machine Learning at Etsy
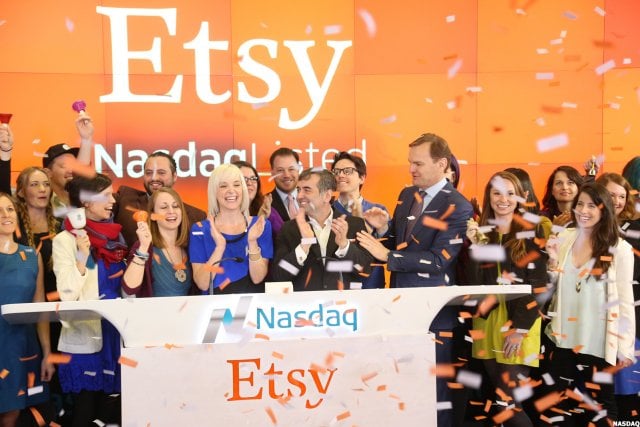

In our latest Real Talk, Aakash Sabharwal discussed machine learning, pioneering context specific search results, industry resources, and all things Etsy. Want to ask Aakash a question? Leave a comment below.
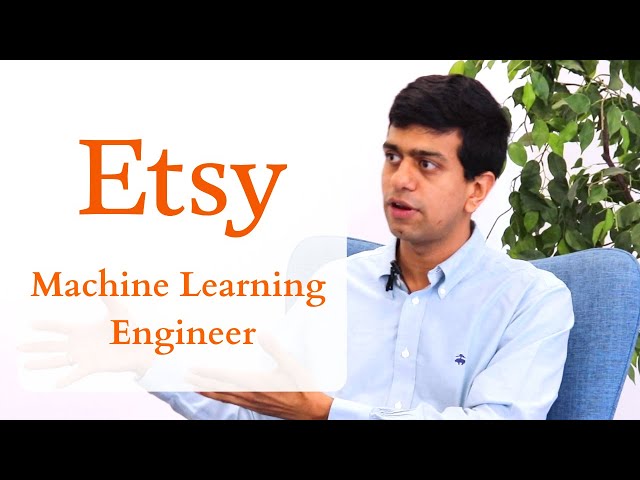
What was your journey to Etsy?
When I first moved to Silicon Valley, I started working on search systems at a startup called Blackbird. Blackbird was a startup with just eight or nine of us providing search solutions to eCommerce companies — allowing different sizes of eCommerce companies to search over their inventory in real time. What’s special about that work was that we were using the images of the listings, image understanding, and computer vision to extract different attributes that helped us serve relevant content.
In 2016, Etsy acquired Blackbird to augment its search efforts.
Since then, I’ve been part of the team helping work on the Etsy search and we have achieved that vision by not only investing in search in different ways, but also growing the data science and machine learning efforts across different product initiatives at Etsy.
What are some of the interesting ML projects you’ve taken on at Etsy?
I was part of the search efforts at Etsy for my first two years — I was leading the project to build the ranking pipeline. What was special was that it was context specific ranking. So the context is what can we extract in real time from the user that helps us make better relevant search results. So this realtime context could be the query, of course – this is entered into the search bar – as well as whatever we know about the user, the time of the day, the device type, the page on Etsy, basically anything in real time that we can extract from the user actions that help us make more relevant predictions.
Get To Know Other Data Science Students
Mikiko Bazeley
ML Engineer at MailChimp
Jonathan King
Sr. Healthcare Analyst at IBM
Haotian Wu
Data Scientist at RepTrak
How are (performance / compensation) levels decided for machine learning engineers?
Levels for machine learning engineers are determined by:
- impact of their work to the bottom line product KPIs — if you build a model, if you build a system, how is that impacting the overall business of the company?
- How are you mentoring others and how are you simply put, helping those around you to grow as well? How are you increasing the surface boundary of your impact beyond just yourself?
- How are you growing technically in terms of the core competency? Are you growing in terms of your machine learning and your applied engineering skills?
If you state a product problem, can you think about what machine learning tools are going to be used? How are they going to break the problem into simple steps, how are they going to incrementally build towards it? And then finally how they’re going to evangelize, get feedback, and improve the system over time. That’s the overall vision and if you improve and execute on those aspects, that’s when you see career growth and project progression.
What are some applications of machine learning that you think have unexploited potential?
Focusing on Etsy, I think marketplace businesses are unique because marketplace businesses have a demand-side and a supply-side – so there are many optimization problems to be solved.
You’re essentially matching the curiosity of a buyer who has varied interests with the offerings of the sellers. There are, of course, the traditional problems of search recommendation, compositional advertisement, those have been the so far the three major focuses at Etsy in terms of machine learning.
Going forward I see that there are problems everywhere. There are problems around how do we predict your style – like what does rustic mean to me? What does modern mean to me? Style is an extremely personal problem and that’s just one symptom.
What may excite you about a listing is very unique to you and it’s hard to figure that out. When you search for coasters on Etsy, you’ll find 250,000 items — functionally, they’re all coasters, they’re all relevant. But for different users, it might be different things. It’s a massive search e-commerce problem with a heavy component of personalization. On top of that, if you add in different machine learning constraints like marketplace constraints, then the problem becomes even more interesting.
Other problems are:
- Optimizing for diversity across sellers — wanting to make sure that all our sellers have a fair chance to be surfaced in the search results to grab impressions
- Free shipping — you may find an item that you like, but because the shipping costs are too high, you may not find it relevant. So how do we weigh those additional costs in?
To summarize, we’ve only scratched the surface with search advertisement and recommendations, but given the constraints of the marketplace, the different growth opportunities, and different ways to personalize in terms of style or functional aspects or taste, I think there’s a huge open space – open canvas – for different applications of machine learning.
If you’re unfamiliar with Etsy, it’s a popular online marketplace for buying, selling, and collecting exclusive and unique items. Etsy was launched in 2005 and has been continuously growing since then. Etsy also has a lot of fascinating opportunities for developers, data scientists, and machine learning engineers.
Since you’re here…Are you a future data scientist? Investigate with our free guide to what a data scientist actually does. When you’re ready to build a CV that will make hiring managers melt, join our Data Science Bootcamp that guarantees a job or your tuition back!