Springboard Mentor Spotlight: Amal Feriani
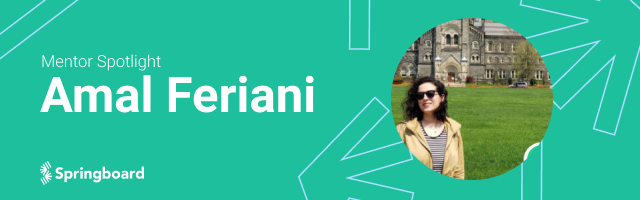
Meet Amal Feriani, ML Research Engineer at InVision and a mentor for Springboard’s Machine Learning Engineering Career Track.
Amal Feriani is a self-taught machine learning engineer. Relying on a mix of online resources from video tutorials to virtual courses, Feriani created her own piecemeal “curriculum” to teach herself the fundamentals of machine learning and coding. Now that she’s a mentor at Springboard, she enjoys being a cheerleader for her mentees and helping them feel less overwhelmed—providing them with the mentorship she never had.
Feriani has worked in various fields from petroleum engineering to game theory and environmental economics. Prior to her current role, she was part of the R&D team at Netatmo, a smart home device manufacturer, where she optimized deep face recognition algorithms for identity verification. Before that, she worked as a data scientist at IBM, where she implemented chatbots using natural language processing.
This conversation has been lightly edited for brevity and clarity.
What do you do at InVision?
I’m an ML research engineer on the AI team at InVision AI. Our objective is to deploy ML models on edge devices (an edge device is any piece of hardware that controls data flow at the boundary between two networks, such as a router).
How long have you been a mentor at Springboard?
I’ve been a mentor for two years.
What do you like most about being a mentor?
I joined Springboard because I myself was mostly self-taught. I learned machine learning from a lot of online resources. When I heard about mentoring opportunities at Springboard, I was excited because I could participate in helping people understand machine learning and help them become machine learning engineers.
The world of machine learning is so attractive—a lot of people want a career in this field—but it can be overwhelming. So to have a good curriculum that captures the key fundamental ideas in machine learning is important. I believe that is what Springboard is trying to do—to deliver a curriculum that builds a strong foundation for ML. Mentors with industry experience help put things in perspective for students. Whenever you face difficulties or challenges, that person can tell you that it’s just a part of the learning process. That is what I try to do with my mentees—I tell them about my own experience in order to help them understand that it takes time to fully understand every aspect of machine learning.
Get To Know Other Data Science Students
Lou Zhang
Data Scientist at MachineMetrics
Haotian Wu
Data Scientist at RepTrak
Sam Fisher
Data Science Engineer at Stratyfy
What is something you have learned from your mentees?
There are a few things. What I like most is my mentees come from different backgrounds, so they bring new perspectives on problems that can be solved using machine learning. This helps me learn about other applications of machine learning.
The second thing I’ve learned is how to convey information. There’s a difference between understanding something yourself and teaching another person the same thing. Being a mentor at Springboard is a good way to challenge myself. Sometimes my mentees ask questions I hadn’t thought of before, which makes me think about ML techniques in a different way. Every interaction with my mentees helps me to deepen and strengthen my knowledge even more.
Can you give an example of a time when you learned something from one of your mentees?
My last mentee had experience designing patterns. She wanted to apply computer vision to create a system that does automatic keywording for different patterns. Keyword tags help people search for photos on sites like Shutterstock. For example, the algorithm sees a pattern and assigns a category, such as floral or geometric. I would see certain patterns and they would look exactly the same to me, but they weren’t. She had artistic knowledge about the different patterns, so her project was actually a fun application of computer vision—how to automatically tag and categorize different patterns online.
How has being a mentor helped your own career development?
Career development for me is really tied to the skillset you develop. Since ML [technologies] are developing at high speed, mentoring has helped me stay up-to-date. Also, helping mentees is both a personal and professional endeavor. You’re not only looking out for your own career; you try to share your knowledge and contribute to developing the community. That is something positive that I want to have in my career. Also, most of my mentees are women, so I feel it is a great opportunity to empower women and help them integrate into the engineering and machine learning fields.

When it comes to training machine learning models, there’s a concept of “garbage in, garbage out,” meaning that the quality of a dataset can perpetuate and reinforce biases. Have you had any experience with this in your career?
I think this is a very important subject to work on, especially for the research community. In a previous role, I worked on facial recognition technology. The racial biases in this field are widely known. My company’s mission was to reduce bias and make the product useful for all genders and races.
What is the most interesting project you’ve worked on in your career?
My first project was the most rewarding. As I mentioned earlier, I didn’t have a diploma in ML, so I had to educate myself. So when I started my first job in ML, I was able to apply something I had learned in the industry to a customer-facing product, which was very rewarding for me. I worked on a lot of different applications of computer vision and machine learning. Computer vision has many challenges, especially when you try to incorporate it into a small device like a mobile phone or something that runs on batteries. My first project was meaningful to me because I made a significant impact on the company’s product.
What advice would you give to someone who is just starting out as a Springboard mentor?
Get to know your mentee’s interests and career ambitions. Beyond that, get to know them personally. For each mentee, I try to ensure the discussion is aligned with their mission and personal interests. My mentees tell me they like that [approach]. They enjoy discussing different possible applications of machine learning towards something they’re passionate about.
And I think what my mentees like is having this glimpse into their future career. I try to explain to them what it’s really like to become a machine learning engineer and share details about my daily process and how I solve problems.
One piece of advice I have is to break the ice so your mentees are comfortable talking with you and asking questions.
What’s your advice for students who are experiencing impostor syndrome?
It’s okay to feel overwhelmed. I’ve been there. You cannot know everything from the [get-go]. You need to go over the material several times. One of my previous mentees said something like, “I can’t do this anymore, this is impossible.” So as her mentor I tried to show her the progress she was making. I told her, “Look, you did all these things. You didn’t know about this machine learning technique before but now you are actually training and getting hands-on experience. This is progress.” And she graduated and did a great job!
All of us go through these phases where we feel like there’s too much to do and not enough time. So I think a mentor’s job is to help mentees feel less overwhelmed because there are a lot of things to cover in machine learning.
What is something new and exciting happening in the field of machine learning?
The most exciting thing about working as a machine learning engineer is you get to bridge the worlds of machine learning and artificial intelligence. We also try to create a bridge between the research community and the industry. We try to bring theories into practice, which can be very challenging. You need to solve a lot of problems, which is actually a very exciting part of the job.
Since ML is developing very rapidly, that means you are always learning and there are always good projects to work on.
What do you like about the curriculum for Springboard’s Machine Learning Engineering Career Track?
The curriculum has evolved quite a bit since I joined Springboard. The first version was thorough and dense, and it covered all aspects of machine learning. Being an ML engineer means you also need to know how to code and solve theoretical problems as well as practical problems. But I think it was a little dense for a six-month course. The newer version of the curriculum [is more concise], with a lot of hands-on experience. You don’t only [listen to lectures] and watch videos—you also work on projects to apply what you learned.
The capstone project is a wonderful opportunity for mentees to apply ML techniques in a field they are comfortable with, and that hands-on experience is crucial. Students then have a project portfolio that helps them find a job after they graduate. So I think Springboard is doing a great job delivering a complete curriculum including ML and engineering.
Springboard mentors have many opportunities to influence the curriculum and provide feedback. Were you involved in designing the new curriculum in any way?
I wasn’t directly involved in creating the new curriculum, but my feelings about it were shared by the community. The curriculum was dense and challenging to finish in just six months, even though it was very good. But with the capstone project and all the learning materials, it was challenging for some of my mentees. So that’s why I think the newer version of the curriculum is much more doable, and all of my mentees have successfully completed the course since we revamped the curriculum.
Data Science and Machine Learning technologies are constantly growing and causing significant technological revolution, as a result, data scientists, data analysts, and machine learning engineers are in high demand as mentors.
Want to become a mentor? Apply here!
Since you’re here…
Curious about a career in data science? Experiment with our free data science learning path, or join our Data Science Bootcamp, where you’ll only pay tuition after getting a job in the field. We’re confident because our courses work – check out our student success stories to get inspired.