Data-Driven Design: What It Is and Why It Matters

In this article

The process of creating a user-friendly product must be guided by information about what works and what doesn’t. Features that look good on paper may end up being impractical or confusing, preventing users from achieving their desired outcomes. That’s where data-driven design comes in.
Data-driven design can be defined as “design that is backed by data and helps [users] understand the target audience.” It “proves that your work is on the right track… reveals the users’ pain points and opportunities while unearthing new trends, and… improves your designs by adding objectivity.”
Here, we’ll cover everything you need to know about data-driven design. You’ll learn which types of data should be prioritized and what kinds of techniques can be used to collect data. We’ll also explore some case studies showing where data-driven design has succeeded.
Why Should We Care About Data-Driven Design?
Failing to consider data (or using data in an ineffective way) can have serious implications for the success of a project. If you rely solely on instinct or best practices to make decisions without performing any data-driven investigation, you risk wasting money on changes to design choices that are ineffective (or even harmful).
Using data effectively can lead directly to improved business outcomes. Research by MIT’s Center for Digital Business found that “companies in the top third of their industry in the use of data-driven decision making were, on average, 5 percent more productive and 6 percent more profitable than their competitors.”
There are many examples of cases where data-driven UX techniques have delivered a tangible improvement on ROI. For example, in 2014, airline Virgin America used A/B testing to redesign a new, responsive website. This led to:
- A 14% increase in conversion rates
- 20% fewer support calls
- Customers booking nearly twice as fast, across devices
Another interesting example comes from e-commerce website Music & Arts, which used usability testing and heuristic evaluation to inform a website redesign. Upon the conclusion of the project, their online sales increased about 30% year over year.
Data-Driven Design: Onboarding Stakeholders
As a UX designer or aspiring practitioner, you may be used to thinking quite a bit about data-driven product design, and may already be convinced of its merits. However, in order to successfully execute your project, you will have to bring many different members of your team on board as well. Below are some tips for presenting data to stakeholders.
Use Case Studies
When introducing new information to stakeholders, you may want to leverage case studies like those mentioned above to show the ROI value of data-driven UX research. With this clear proof of effectiveness, you can demonstrate value in a concrete way.
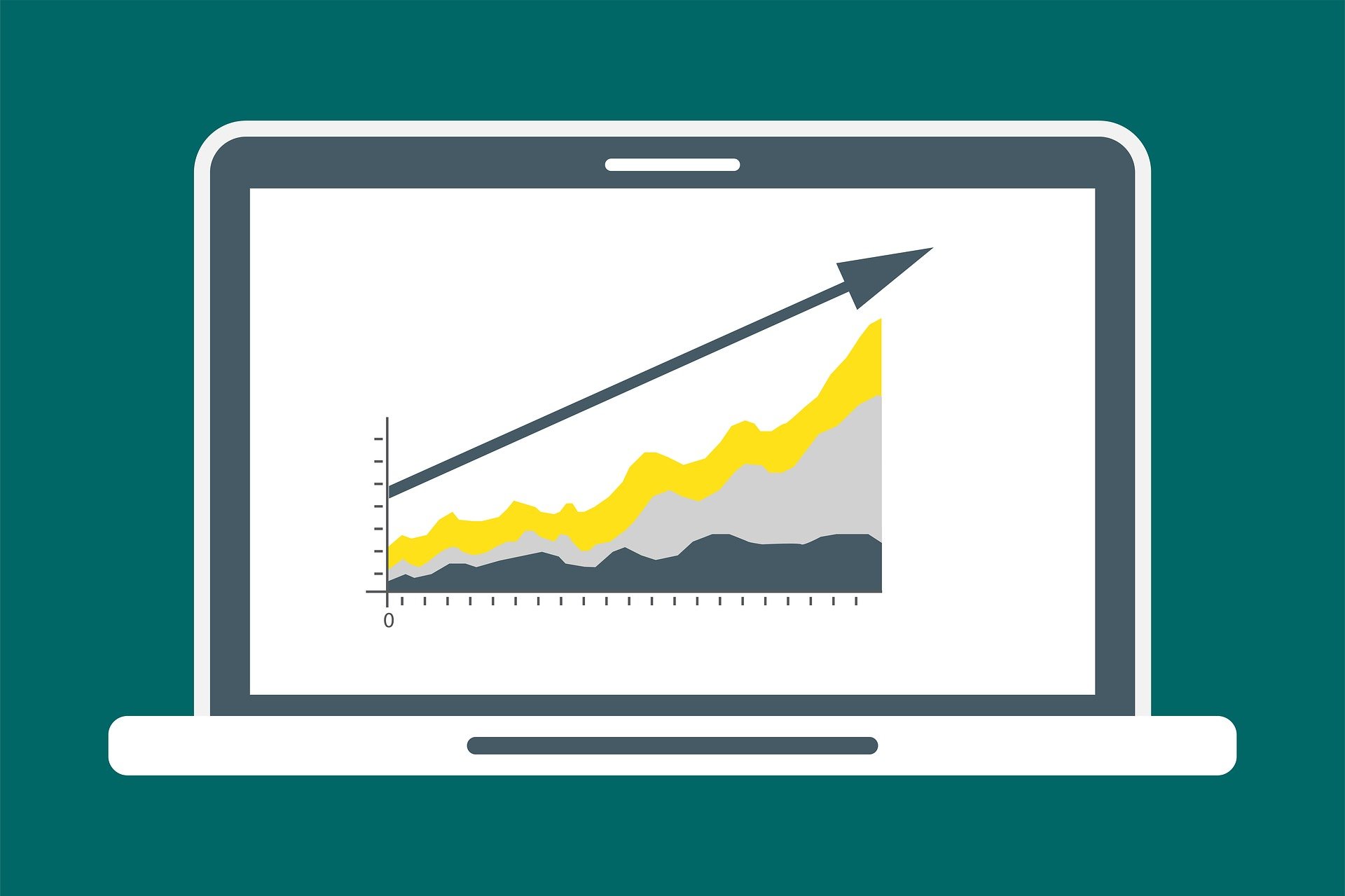
(Source: Pixabay)
Present Data Visually
Visuals are convincing. In fact, a 2014 study revealed that over half of all respondents considered themselves to be at least partially “visual learners.”
In the marketing world, the use of visuals has become a popular way to stand out and capture attention—80% of social media marketers report using visual assets as part of their strategy.
By adding visuals to stakeholder-facing presentations, you can captivate your audience and clearly convey your message. A slide deck with graphs and charts can go a long way.

(Source: Wikimedia Commons)
Data-Driven Design Thinking: An Important UX Philosophy
Once you’ve gotten your stakeholders on board, it’s time to start thinking about collecting data. But how should you go about doing this?
Data-driven design thinking isn’t just about gathering as much data as possible. Ideally, you’ll want to develop a plan for collecting data in accordance with your UX and business needs.
Establishing Realistic Goals
While data is an important part of the UX design process, it’s not the only factor in decision-making. You’ll have to consider feasibility, cost, timing, and other factors when applying the information you have gathered.
For example, perhaps your product has already launched, and you’re looking to design a new iteration of it with some improvements. Your data-gathering process will look very different than that of someone creating a new product from scratch. It might not always be realistic or cost-effective to overhaul your entire product or business concept.
Ideally, you’ll want to make data-driven decisions right from the very beginning. But realistically, you’ll need to consider the unique needs of your organization when deciding how data can help you.
Design Ethics
When developing designs to test, you’ll want to consider reputation, branding, and ethics, as well as quantitative results.
For example, using hidden fees or misleading links on a website might cause those options to perform well in the testing phase. However, by using those kinds of tactics, you risk alienating your target audience and damaging your brand’s credibility, leading to a reduction of ROI in the long run.
The short-term gains may not be worth the long-term risks.
Data-Driven, Data-Informed, and Data-Aware Design
In the book “Designing with Data” by Rochelle King, Elizabeth Churchill, and Caitlin Tan, the authors present a layered model of “data-driven,” “data-informed,” and “data-aware” design. A UX Collective article by Aaron Gitlin further explains this model.

(Source: Screenshot, UXdesign.cc)
The model describes three different philosophies for using data in UX design work.
Data-Driven Design
By King, Churchill and Tan’s definition, “data-driven design” refers to making design decisions based solely on quantitative data. Within this framework, data is of primary importance.
A purely data-driven approach may be appropriate when the main goal of the project is performance optimization.
Data-Informed Design
“Data-informed design” is a somewhat more flexible approach. In this case, additional factors such as qualitative information, instinct, and experience might be prioritized, alongside quantitative data.
Data-Aware Design
A data-aware design team would put quantitative data on an equal footing with other decision-making factors. This kind of team views data from UX testing as just one of many potential sources of valuable information.
When deciding which of these three approaches is right for you, it’s important to consider the individual dynamics of your team, as well as the circumstances of your particular project.
Get To Know Other Design Students
Sadie Rubio
UX Designer And Researcher at Lumea
Aisha Butt
UX Associate at Meta
Amber Hicks
UX Designer at Norfolk Southern
Data-Gathering Procedures: Best Practices
Now that you’ve planned how to use your data, it’s time to conduct tests to gather the hard numbers you need. Below, we’ll discuss the process of planning for a UX research experiment.
Creating a Hypothesis
In the previous section, we spoke about establishing goals for your project (what will your data eventually be used for?). Once you’ve done this, you can turn your attention to developing a hypothesis.
Creating a hypothesis for a UX experiment is much like creating one for a science experiment. Many of the same rules apply.
The biology department at California State University, Bakersfield, has this to say about hypotheses (emphasis theirs):
“A useful hypothesis is a testable statement, which may include a prediction. A hypothesis should not be confused with a theory. Theories are general explanations based on a large amount of data.”
They go on to give the following example of a “formalized hypothesis”:
“If skin cancer is related to ultraviolet light, then people with a high exposure to UV light will have a higher frequency of skin cancer.”
The statement has two parts: If X condition is met, then Y result will occur. It refers to a cause-and-effect relationship between two factors.
When creating a UX hypothesis, however, it’s necessary to go a little bit further. Beyond just discussing the cause and effect, we need to explain which users our hypothesis applies to, and why we think the result will occur.
An example of a formalized hypothesis for a UX research project can be seen below:
If the color red creates a sense of urgency in users, then making the “checkout” button on our website red will increase conversions among users browsing our product pages.
As you can see, the UX hypothesis is slightly longer than a scientific hypothesis. It contains all the information we need to test and answer the question.
Ensuring a Sufficient Sample Size
Once you’ve created a hypothesis, you’re ready to begin your experiment.
When conducting an experiment, there are certain best practices to keep in mind. One is that you need a sufficient sample size to ensure results are significant.
If your sample size is too small, the value of any data you gather will be questionable. For this reason, it’s important to ensure that your organization has a method of finding and incentivizing UX testers to participate in the experiment.
Eliminating Confounding Variables
You’ll want to eliminate confounding variables as much as possible when planning your experiment.
For instance, in the above “red button” example, it might not be a good idea to redesign your entire website, then test the old version of the website with the former button color and the new version with the red button.
In that case, users might be tempted to purchase because the new overall design makes the website look more trustworthy—the results could have nothing to do with button color.
UX Data Collection Techniques
There are many different UX techniques you can use to collect both qualitative and quantitative UX data. Below are just a few examples:
Quantitative Data Collection
A/B Testing
A/B testing is also known as split testing. Hubspot defines A/B testing as:
“… [an] experiment wherein you ‘split’ your audience to test a number of variations of a campaign and determine which performs better. In other words, you can show version A… to one half of your audience, and version B to another.”
When performing an A/B test, it is important to ensure that only one variable is changed (whenever possible), and that the control and experimental groups are similar in size.
Related: 8 Common A/B Testing Mistakes You Should Avoid
Surveys
UX surveys are a key source of both quantitative and qualitative data in UX research.
A good survey should have well-designed questions—ensure that your questions are not leading and that the purpose of the question is clear. You should also try to limit the number of questions (no more than 10 to 15) so that users don’t abandon the survey halfway through.
Analytics
If your product is a website or app, tools such as Google Analytics are a great source of quantitative data (click-through rate, bounce rate, etc.) to help you make decisions.
Heat Maps
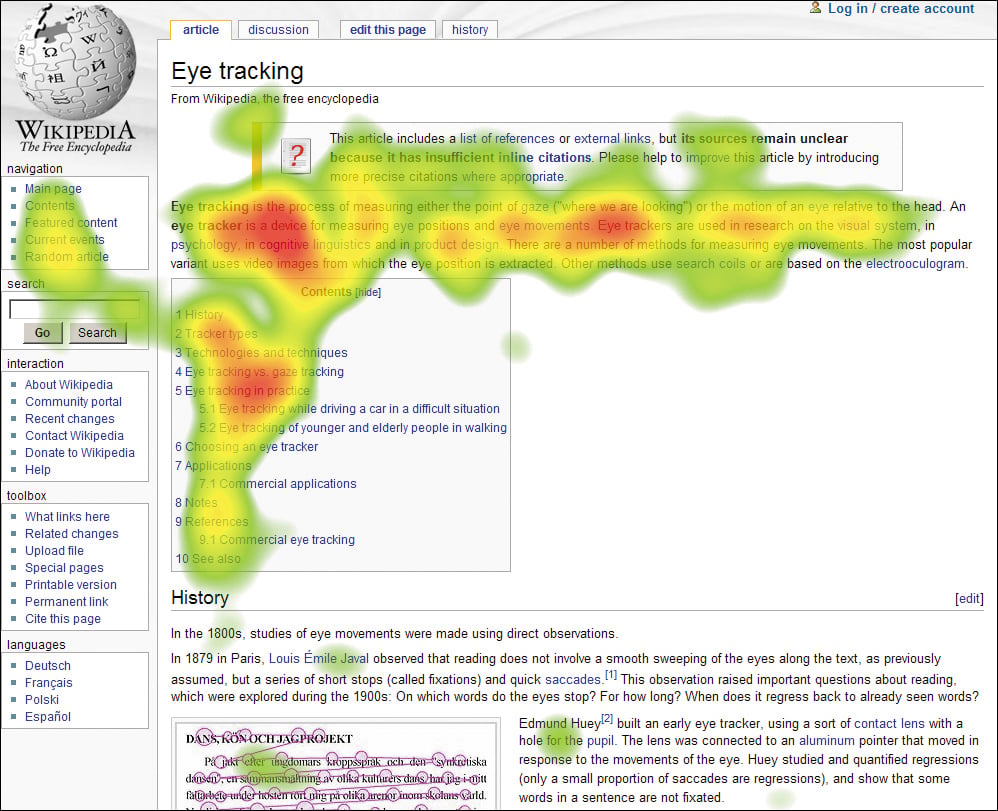
(Source: Flickr)
Heat maps use eye tracking to understand where users are looking on a screen. If heat maps from multiple users indicate a pattern, this could prove valuable when re-organizing content assets or redesigning your website or app.
Qualitative Data Collection
Competitor Analyses
In UX, a competitor analysis involves examining another company’s product to identify any comparative strengths, weaknesses, or areas for improvement.
It is important to tread carefully when doing a competitor analysis. Simply imitating competitors is not always an effective solution. Instead, it is best to use competitor analyses as a means of gaining inspiration, with the understanding that what works for others may not always work for you.
Interviews
Interviews are a great way to gather qualitative data from users. Although time or budgetary constraints might limit the number of interview subjects, the insights gathered through a phone or in-person conversation will be more in depth than what you could get from a survey alone.
User Journey/User Flow
Creating a model, such a user journey or user flow, can be a helpful way to conceptualize how users are interacting with your product. The information you gather from your user flow can help you identify potential weak areas, providing a starting point for further investigation through A/B testing or interviews.
Related: 16 Important UX Design Principles for Newcomers
Regardless of your goals, data-driven design can help improve product performance and increase conversions. Hopefully, the information presented here can help you create an effective plan for data collection that fits your organization’s needs.
Since you’re here
If you want to work in design, you can. It’s that simple. With our UX Design Course, you’ll launch your career in design in 9 months or less – guaranteed. Browse our free UX salary guide to see what you could be making