How To Build an Awesome Data Science Portfolio (+5 Examples)

In this article
Have you ever wondered why there’s so much emphasis on portfolios—and not on resumes—when it comes to applying for data science jobs? That’s because, given that data science is an emerging field, hiring managers often care more about the work you do than the number of years of experience that you have.
But what does a data science portfolio actually look like? Data visualizations are certainly part of it, but there’s much more to include than you might expect.
That’s why we’ve created this guide. Below, we’ll outline some simple steps to help you create a winning portfolio, and share some excellent examples of data science portfolios you can draw inspiration from.
What Is a Data Science Portfolio?
A data science portfolio is a collection of your best work and demonstrates your skills as a data scientist. A data science portfolio should feature a combination of your code and documentation and some writing samples showing your ability to communicate effectively about data. They also help visualize your process and how you think about problem-solving.
Why Do You Need a Portfolio?
A data science portfolio will highlight your expertise in deep learning, exploratory data analysis, computer science, programming languages, and more. It can also be a great way to showcase any science projects or personal interests related to data science—like creating an app or website or working on data sets at home.
Elements of a Great Data Science Portfolio
Here are four elements that every great data science portfolio needs.
Concise Explanation About Yourself and What You Do

You should create your portfolio in a way that clearly and concisely describes who you are and what you do. It’s not enough to say, “I am a data scientist” or “I am an AI engineer.” You need to describe yourself in terms of your communication skills, experience, and accomplishments.
This is also where you should talk about any project samples relevant to your field. For example, if you are applying for a job as a startup data scientist, it makes sense to include any projects related to startups in your portfolio.
Quick Load Time
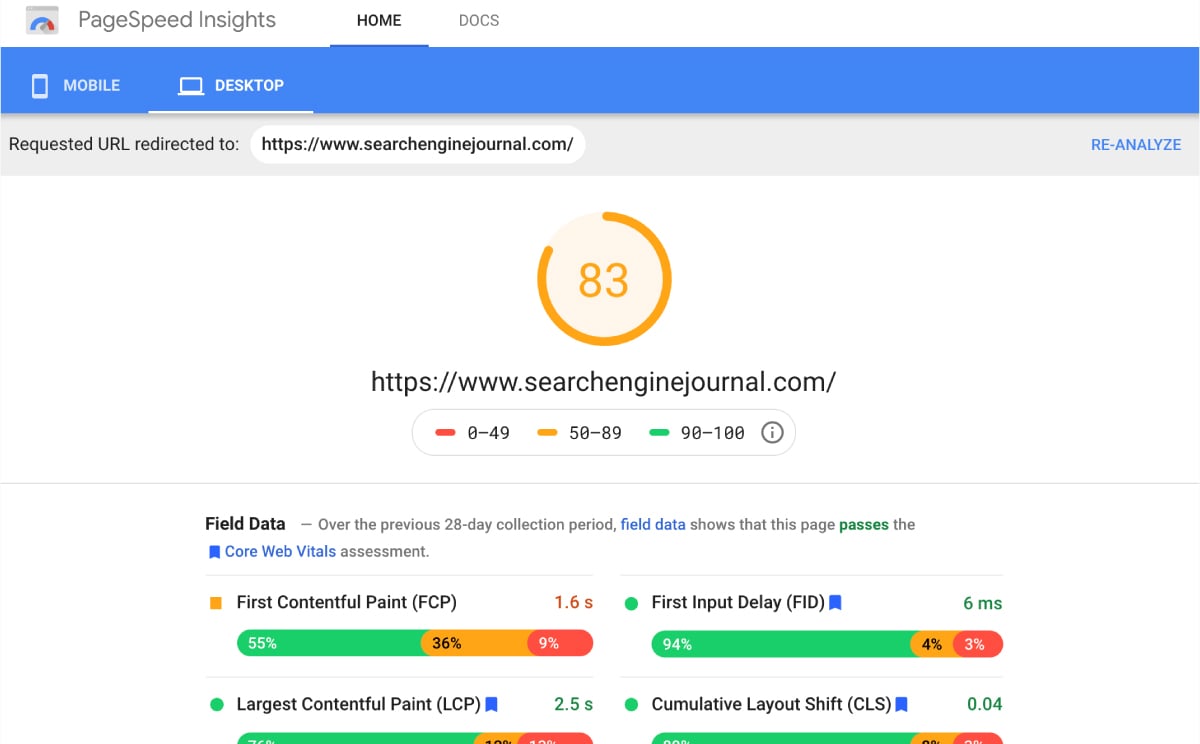
Website speed is critical—you want to ensure that your website loads quickly, so viewers don’t decide to click away before they get to see your work. You can use Google PageSpeed Insights to test how fast (or slow) your site loads on different devices and browsers. If necessary, hire someone to optimize your website’s load time if it’s too slow.
Professional Design

Your portfolio should look professional, unlike a personal blog or resume. It should be easy on the eyes and display information about you and your work without distractions like ads or pop-ups that could distract users trying to navigate the site.
Illustrates Your Best Work and Projects
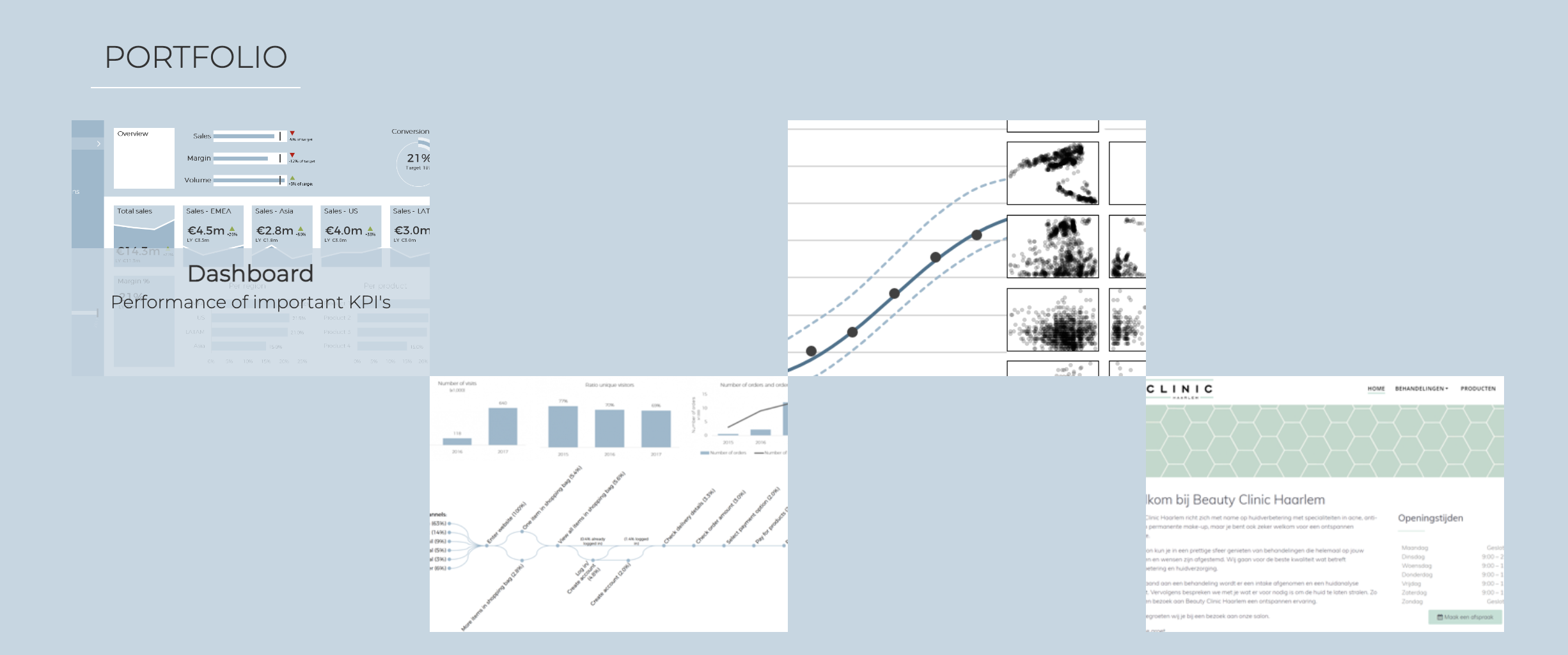
Your portfolio should illustrate all of your best work and projects in an easy-to-understand format that’s easy on the eyes and user-friendly.
A portfolio with too much information will make it difficult for recruiters to find exactly what they’re looking for quickly, so keep it simple.
Social Proof
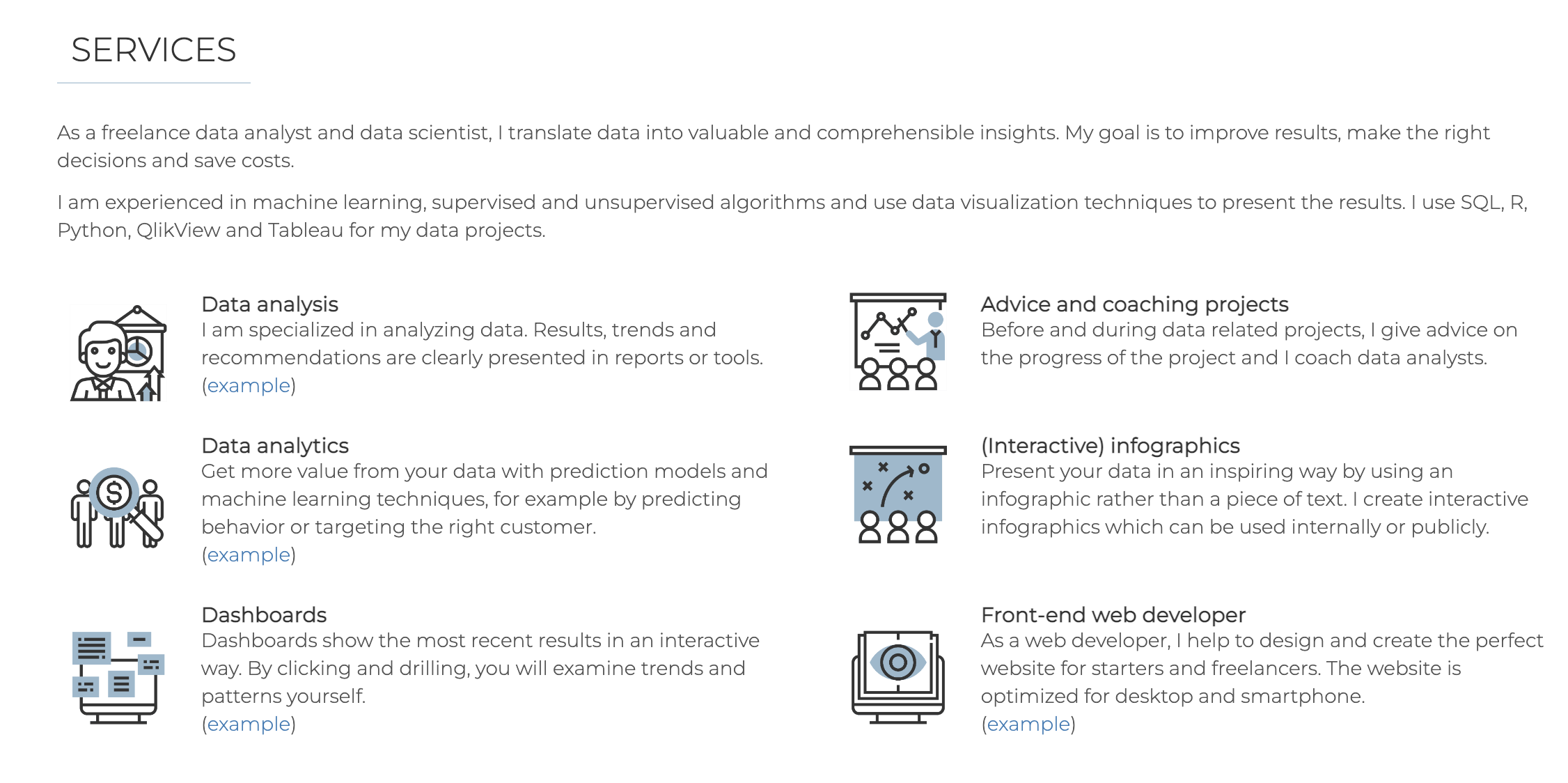
If you’re going to be a data scientist, you need to be able to prove it—and the best way to do that is with social proof. This could be an impressive list of accomplishments, or it could just be a few lines describing why the people who matter to your career think you’re one of the best.
A Straightforward Way To Reach You
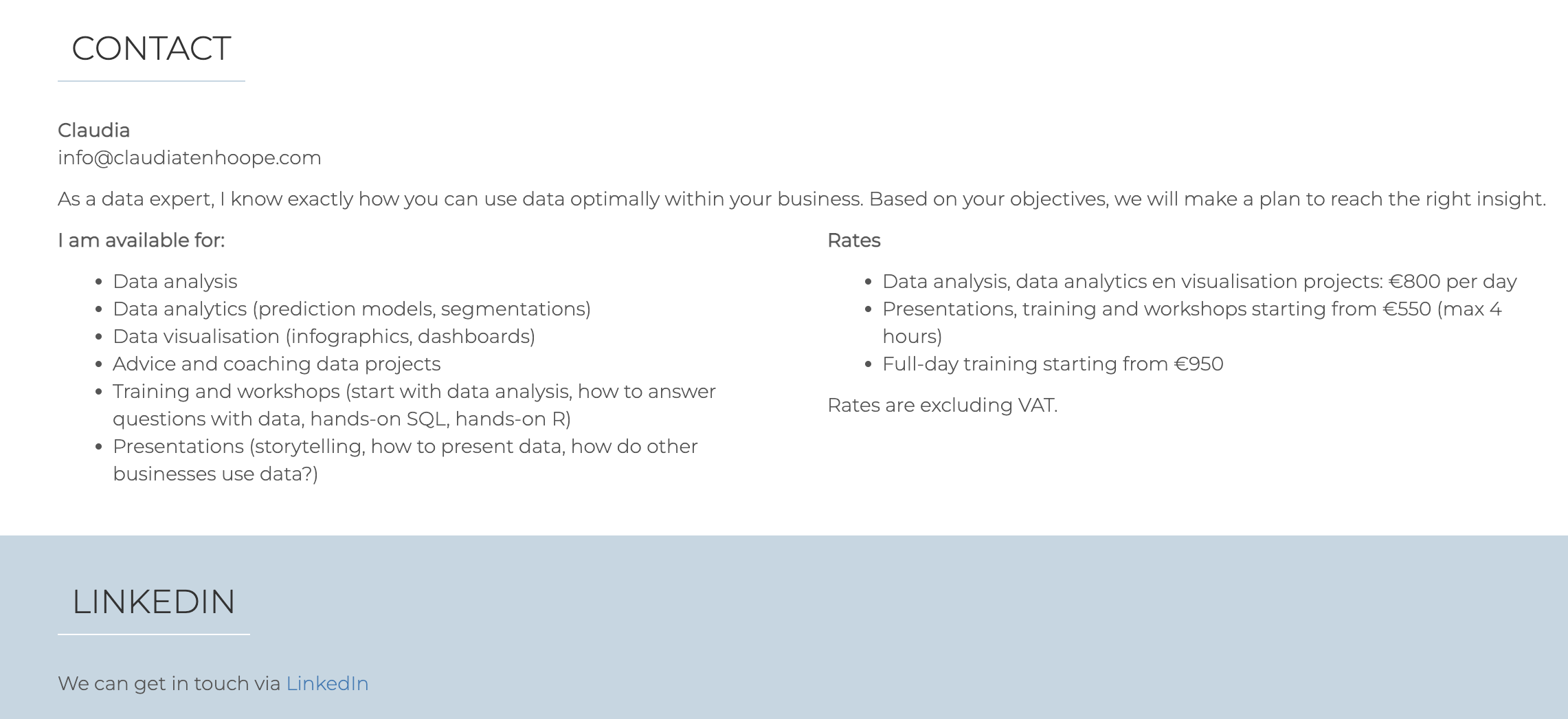
Make it easy for people wanting to hire or collaborate with you to get in touch. If someone wants to reach out, they should have an easy way to do so without going through too many hoops.
How To Build Your Data Science Portfolio
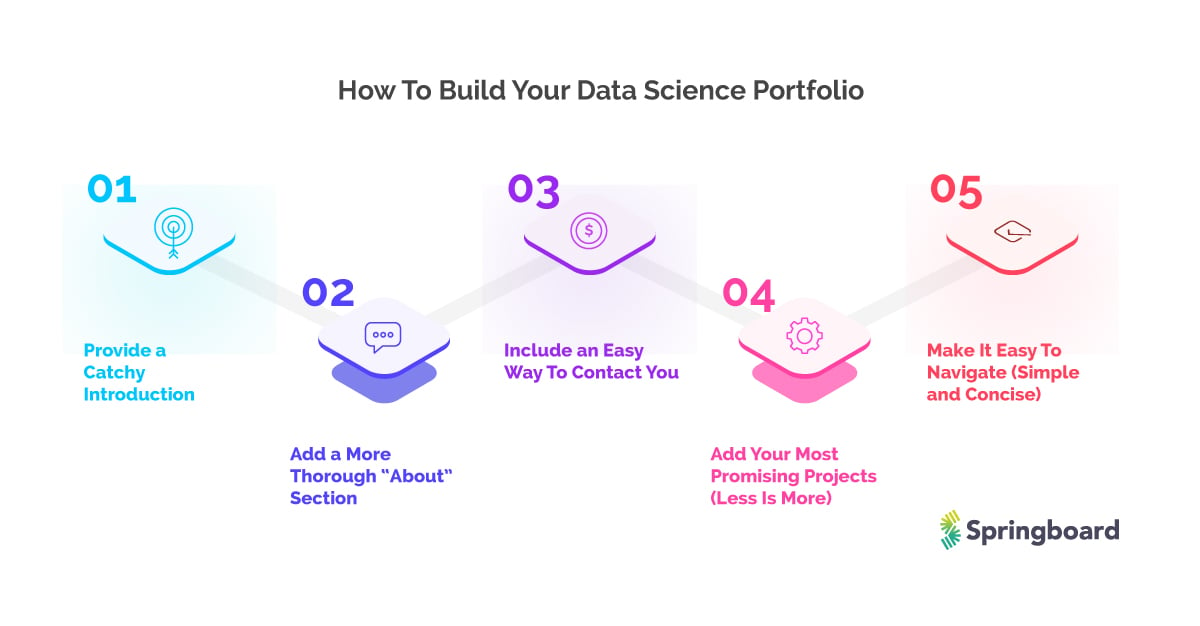
-
Provide a Catchy Introduction
-
Add a More Thorough “About” Section
-
Include an Easy Way To Contact You
-
Add Your Most Promising Projects (Less Is More)
-
Make It Easy To Navigate (Simple and Concise)
-
How To Stand Out
-
What To Avoid
If you’re ready to build your portfolio, but aren’t sure where to start, then here are some tips for how to build your data science portfolio:
Provide a Catchy Introduction
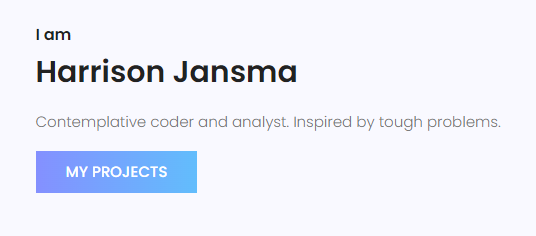
Source: H jansma
You need to make sure your introduction is as catchy as possible. Start with a short sentence that describes your data science skills and experience, then follow that up with a short paragraph explaining how those skills will help your employer achieve the goals of the data science job description.
Add a More Thorough “About” Section
Your “About” section should include information about your education, experience, and any data science courses or bootcamps you have completed. It should also have links to any machine learning projects or publications you have been involved in that relate directly to the position you are applying for.
Include an Easy Way To Contact You
You can do this by providing your email address or phone number. Still, it is also good practice to include social media handles (Twitter or LinkedIn) or even a link to your other websites if applicable.
Get To Know Other Data Science Students
Haotian Wu
Data Scientist at RepTrak
Diana Xie
Machine Learning Engineer at IQVIA
Jasmine Kyung
Senior Operations Engineer at Raytheon Technologies
Add Your Most Promising Projects (Less Is More)
You want to ensure that you’re highlighting your best assets and showing them off in the best light possible. Here’s what you should do:
Highlight Work Done for Prominent Companies in Your Niche
To get an edge on the competition, include projects you’ve worked on for well-known companies in your field. This could consist of writing reports about market trends to developing tools that help CEOs make business decisions.
Focus on Common Projects
These can include building models to predict customer behavior, visualizing data, creating machine learning systems, or cleaning and manipulating data.
Consider Adding a Page or Section for Each Project
If recruiters can see the specific details behind each project, they’ll get an even better idea about which ones are most relevant for them.
Include Project Details, Learnings, and Takeaways
Whatever approach you choose, ensure you include all the information on the project: what kind of problem were you trying to solve, what tools and methods you’ve used, what results you’ve achieved, and what lessons you’ve learned from the experience.
Make It Easy To Navigate (Simple and Concise)

Source: Claudia Ten Hoope
When building your data science portfolio, it’s important to remember that you’re not just showcasing your science skills. You’re also creating an experience for the people reviewing your work.
It’s not enough to just have a bunch of different portfolio projects: You need to ensure that they are easy for an interviewer to navigate and understand. If a reviewer has to spend too much time figuring out what they’re looking at and where to go next, then they won’t be able to focus on what matters most: your expertise.
One of the best ways to make your portfolio easy to navigate is by using a table of contents. This can be as simple as a list of links at the top of your page, or you could use a clickable image that takes users directly to each section of your portfolio.
How To Stand Out
Here are some tips for making your data science portfolio stand out:
Use a Self-Hosted Solution
It’s essential that you host your portfolio on your website, not a freelancer platform. This gives you more control over how it looks and feels, which helps it feel more professional and trustworthy.
Invest in an Appealing Design and Layout
Your portfolio should look sleek, professional, and modern—the kind of website that would make someone want to work with you. If it doesn’t look like that yet, consider hiring a designer to help make it more attractive.
Talk About the Types of Projects That Interest You
Be sure to emphasize any experience working on projects similar to those for which you’re applying—this will show potential clients that their needs are within your scope of expertise.
Illustrate Work Traits That Will Help Potential Clients Get To Know You
Present yourself in a way that is both relatable and professional. To do this, you’ll want to include examples of work demonstrating your strengths as a data scientist and any hard or soft skills you possess.
Document the Tools You’re Familiar With and How You’ve Used Them in the Past
You can accomplish this by creating a list of the tools you’ve used for previous projects and explaining how you used them. Doing so can help potential clients understand what kind of work suits them best.
What To Avoid
When starting out, it can be hard to know what to include in your portfolio, especially if you’ve only got a little bit of experience under your belt.
We think you should avoid including three things in your data science portfolio:
Never Copy Another Portfolio
Your data science portfolio is your chance to show off what you’ve learned and what you’re good at. It’s also your chance to prove that you can think creatively and solve problems independently, so don’t waste it by copying another person’s work.
Never Use Formulaic Website Templates
Your website is your first impression, so you want it to be as unique as possible. The best way to do that? Don’t use a template to create your site. You’ll end up with something that looks generic instead of genuinely representative of who you are and what you do.
Don’t Include Projects You Aren’t Proud Of
If there are projects in your portfolio that don’t reflect well on your skills or interest level in data science, leave them out. You have enough space on the page for only the things that make you look good; don’t waste time trying to shoehorn in an irrelevant piece just because it was one of your first projects ever made with Python.
Data Science Portfolio Examples
Data science portfolios are a great way to show off your skills and expertise as a data scientist. If you’re looking for a new job or internship, it’s essential to put together a portfolio that will impress hiring managers—and get them excited about what you might bring to their company.
But what makes up a good data science portfolio? And how do you make sure it’s clear, organized, and convincing? Here are five strong portfolios to learn from.
Natassha Selvaraj

Natassha’s data science portfolio is well organized and includes a thorough “About” section, a “Projects” section, links to her GitHub portfolio, social media, and relevant certifications.
We love this portfolio because it’s clear that Natassha has thought about what she wants potential employers to know about her. Her “About” section is detailed and gives us an idea of how she thinks and what she wants out of life.

The “Projects” section shows us that Natassha can develop different kinds of projects and explains her process in detail. It also shows us that she’s willing to tackle new challenges head-on.
Finally, the certifications section tells us about some skills or knowledge Natassha has acquired outside her degree program. This helps show us that she’s committed to learning throughout her data science career.
Jessie-Raye Bauer
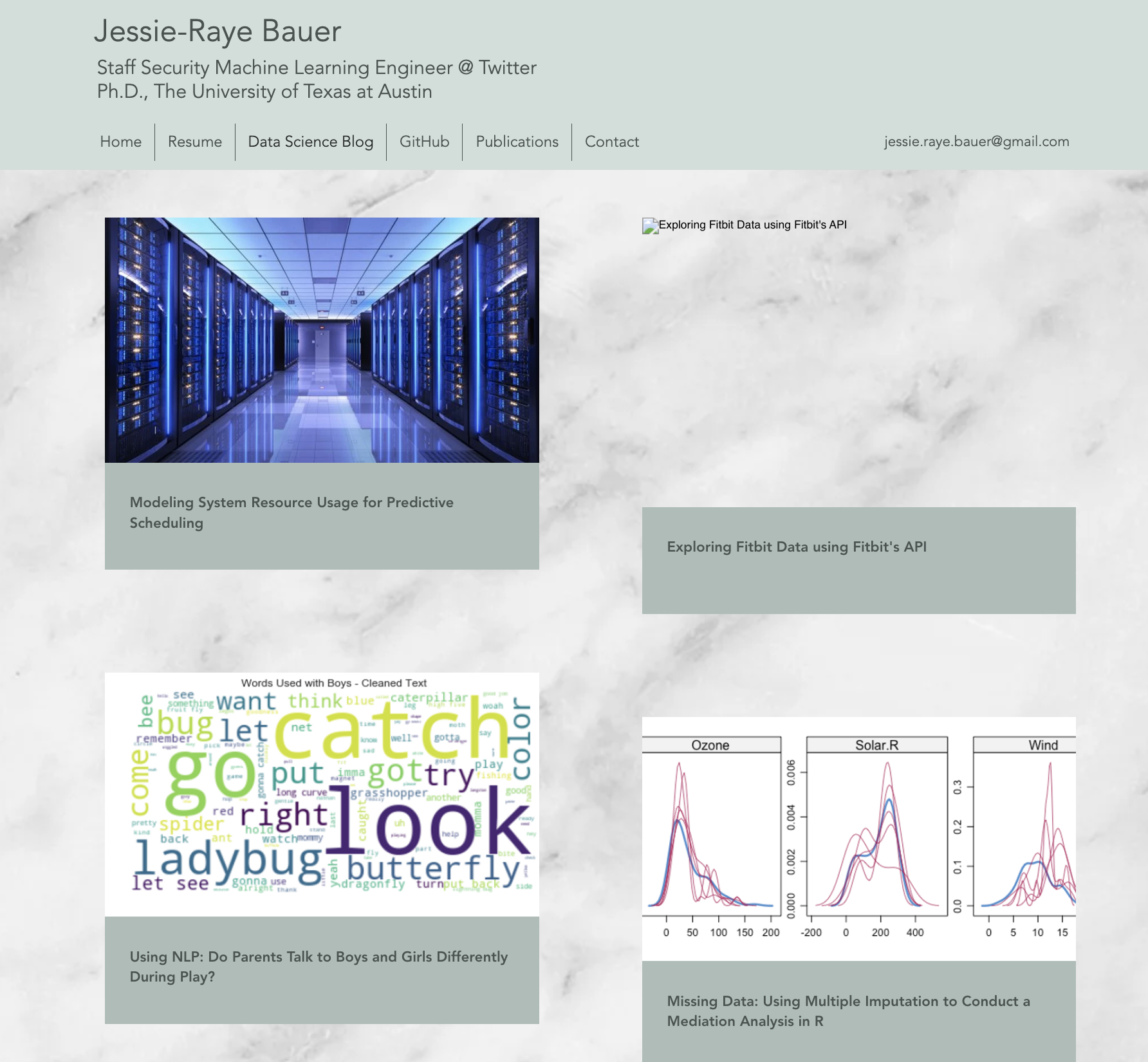
Jessie’s data science portfolio is professional and well-organized. Potential employers also have access to her professional blog and several publications, which clearly demonstrate her expertise. The contents page makes it easy for employers to find what they need, and the organization of each item—the links between them and how they’re written—makes it easy for employers to get a sense of Jessie’s work history, interests, and skills.
We also like that Jessie has included links to her blog posts and publications on this website because they show that she has experience publishing articles about data science topics. It demonstrates that she’s interested in sharing her knowledge with others.
James Le
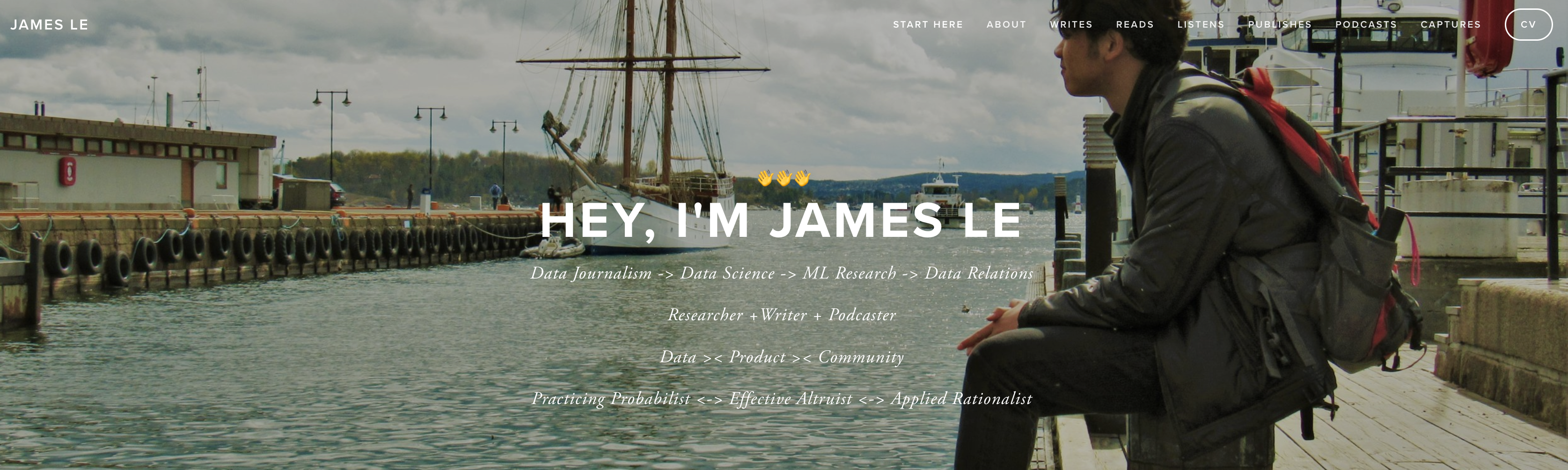
We love how James’ data science portfolio is presented. He does a great job of onboarding potential recruiters by having a “Start Here” section where they can learn about him. This is an excellent way to help potential employers understand who you are and what you’re looking for.

We also like that James shares his favorite podcasts or books on his site and his thoughts on why he’s passionate about data science. This helps employers better understand who he is and what makes him tick, which can help them determine whether he’d be a good fit for their company.
Katie Jolly

Katie’s portfolio is presented as a swipe file that displays all her projects on one page. She linked her resume on her site and also links to her blog.
This is a great way to show off your personal projects because it allows readers to quickly see what you’ve worked on over time instead of having to click through multiple pages. It also allows you to link to your website or blog so they can learn more about who you are and why they should hire you.
Hannah Yan

Hannah has a simple portfolio, showcasing only her most important projects. She briefly explains the projects and then sends those interested to the projects’ GitHub.
We like this portfolio because it’s easy to read and demonstrates Hannah’s technical skills and interests. We also like that she includes links to the projects so potential employers can see what she’s done in greater depth.
What Do Recruiters Look for in a Portfolio?
Experienced data scientists are expected to be able to work with large amounts of data and analyze and process it. But they’re also expected to have a firm grasp of what their employers need. A good portfolio showcases that you understand not just the technical challenges of your job but also how to ensure that your work has a clear purpose and impact on your company. This can include:
- A demonstration of your ability to analyze data using various techniques and tools (such as Python, R, SQL, and Tableau)
- A demonstration of your ability to communicate findings clearly and concisely. For example, you should be able to present findings in a way that would be understandable to someone unfamiliar with the industry or subject matter.
Data Science Portfolio FAQs
We’ve got answers to your most frequently asked questions.
How Do You Present a Data Science Portfolio?
Your data science developer portfolio must show that you have the skills and experience necessary to succeed in this field. To do this, you’ll want to include examples of projects you’ve worked on. You can also include examples of any code or programs you’ve written. If possible, these should be open-source projects so potential employers can view them.
You should also include information about your education and training history and any certifications or relevant degrees you may have earned. Finally, don’t forget about your personal interests. If anything else makes you an especially strong candidate for a position, make sure it’s included in your portfolio as well.
Should You Include a Resume in Your Data Science Portfolio?
If you’re just starting out, having a resume on hand is probably a good idea—it can be helpful to have it when you’re trying to get your foot in the door at companies. But if you have more experience, you might not need it.
The best way to know for sure is by talking to recruiters and hiring managers in your industry. Ask them what they’d like to see from candidates looking for positions like yours, and then do what feels suitable for your situation.
How Long Does It Take To Become a Data Scientist?
Generally speaking, it can take anywhere from six months to two years to become a data scientist. It’s essential to keep in mind that there are many different ways to get there.
For example, if you’re an experienced software engineer who wants to switch to data science and is looking for more formal education, it might take about one year or so. However, if you have no experience with programming or statistics (or any other field related to data science) and want to start from scratch, it could take closer to 2 years.
The good news is that there are many data science bootcamps that can give you the skillset and knowledge necessary for entering this field.
Since you’re here…
Curious about a career in data science? Experiment with our free data science learning path, or join our Data Science Bootcamp, where you’ll only pay tuition after getting a job in the field. We’re confident because our courses work – check out our student success stories to get inspired.