How Can We Fix the Data Science Talent Shortage?
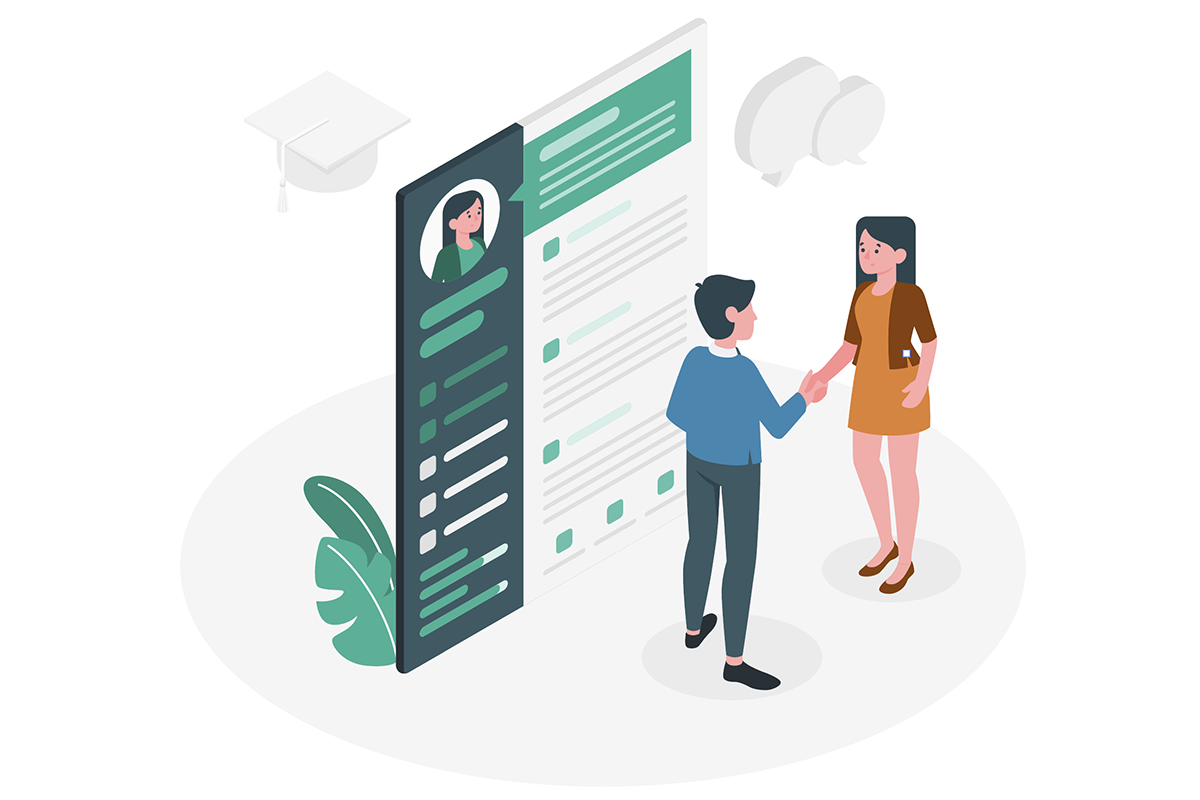
Data science might just be the most buzzed-about job in tech right now, but its pop culture sheen conceals some of the harsh realities of being a fresh graduate in the industry.
The job topped LinkedIn’s yearly Emerging Jobs Report from 2016 to 2019 consecutively (it is now at #3). But when Springboard data science alum Kristen Colley started hunting for her first data science job in 2019, most companies were not interested in her data science credentials. “When I started rebranding myself as a data analyst with the ability to handle machine learning problems, that’s when the opportunities started coming in,” she said.
Colley’s experience is part of an emerging trend in the way companies hire data scientists. With the mainstreaming of automated machine learning (autoML) and DataRobot, an AI platform which can train and tune machine learning models, businesses don’t necessarily need full-fledged data scientists who can perform end-to-end data processing, from exploratory data analysis to building ETL pipelines—at least not for junior roles.
“If you want that high-paying data science job you signed up for, you’re going to have to wait a few years,” said Hobson Lane, a Springboard data science mentor and co-founder of Tangible AI. “They’re moving up the skill level chain because they can now get much of what they need for data science from DataRobot and autoML.”
Entry-level data jobs tend to focus on working with these tools—tuning hyperparameters for machine learning models and cleaning data—rather than building and training machine learning models. Those types of roles, which can have a direct impact on executive decision-making, are largely reserved for data scientists with five or more years of experience.
“Companies are still hiring those people that can solve problems that automation can’t,” continued Lane. “These tend to be people that have a bit of experience in the world working on a very specific problem and they have the expertise that these [tools] haven’t yet incorporated.”
A high barrier to entry
Data science has always had a high barrier to entry, with 42% of data science roles requiring a master’s degree or higher, according to a recent Burning Glass report. As automation takes over the menial parts of a data scientist’s job (80% of a data scientist’s time is spent cleaning data), the bar for landing a data science job is both higher and lower, said Lane—a trend somewhat analogous to America’s shrinking middle class.
Companies seek to fill lower-paying data analyst roles that require fewer years of experience, while also hiring highly skilled data scientists with domain expertise who can solve a specific business problem—like determining risk factors for certain diseases or increasing voter turnout. An analysis by KDNuggets revealed that on average, companies require candidates to have at least 4.2 years of experience as a data scientist and 5.2 years of experience in related fields.
Colley advises new grads seeking a data science role to find work as a data analyst but then to offer to help the company tackle machine learning problems to get real-world experience in that domain. “You’re now a data analyst that can deal with any business problem that comes up, whether they have it in their mind right now or in the future,” she said.
After gaining a few years of work experience in data analysis while solving problems with machine learning, you’ll have an easier time landing a pure data science role.
Even if it’s hard for data science graduates to land their dream job right off the bat, it’s still a promising field as the demand for data scientists continues to skyrocket. The Bureau of Labor Statistics predicts that data science roles will grow 15% from 2019-2029, much faster than the average for all occupations, while the average base salary for a data scientist in the U.S. is $122,582, according to Indeed.
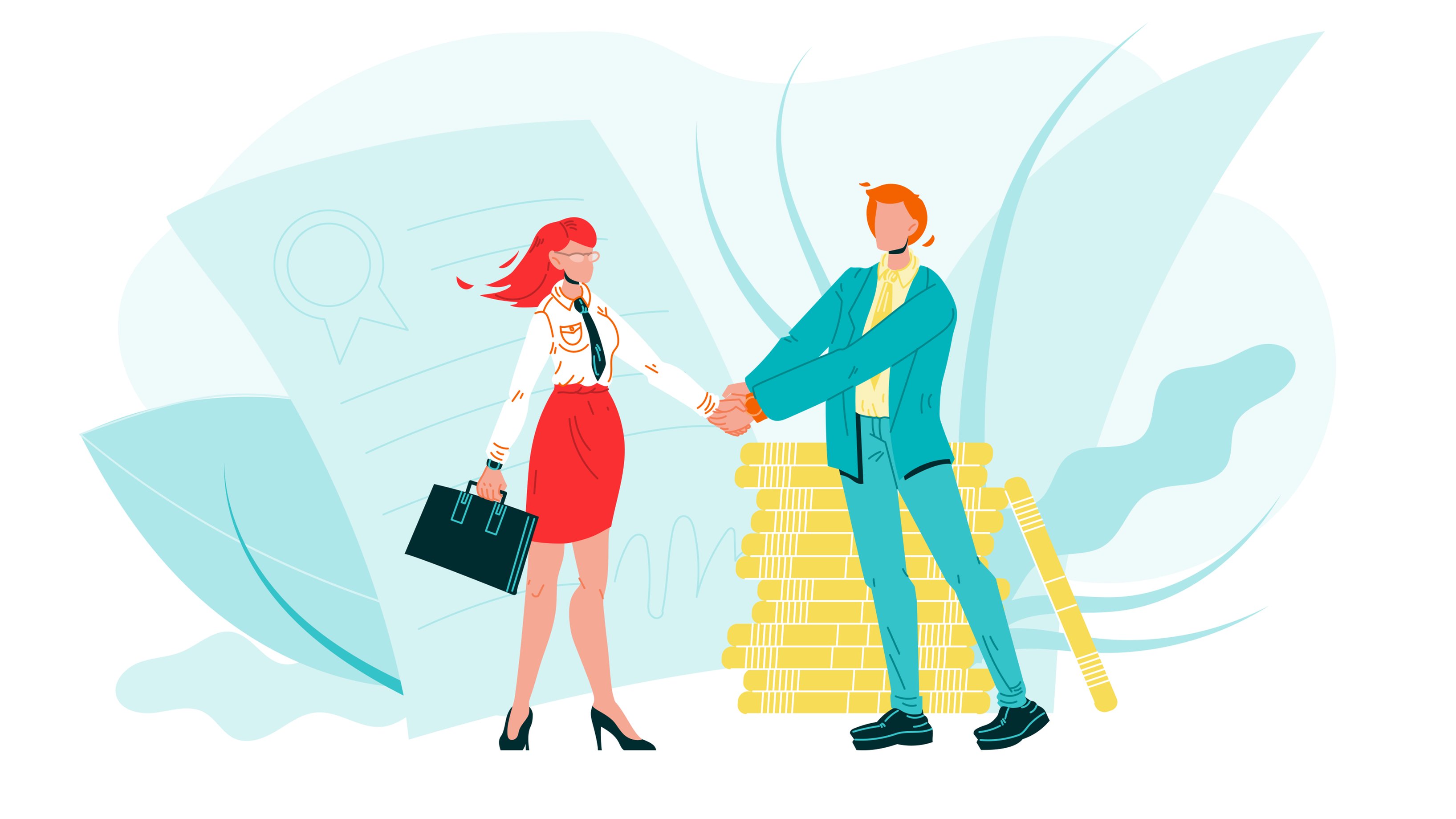
Why are data science roles in such high demand?
As the world goes digital, the amount of data we generate is growing exponentially, to the tune of 2.5 quintillion bytes every day. The bulk of this comes from the Internet of Things (IoT) devices. In fact, the coronavirus pandemic has ushered in new types of wearable technologies that log biomarkers like skin temperature and breathing rate as a proxy for detecting symptoms of COVID-19. Some sports leagues, factories, nursing homes, and universities are already using them to predict virus exposure.
When government-mandated lockdowns went into effect towards the beginning of the year, consumers changed their habits en masse, turning to streaming services, online shopping, telehealth, and contactless mobile payments. This spurred unprecedented growth in data-generating services. Nearly one-third of U.S. consumers used contactless payments for the first time since the pandemic, the majority of whom plan to continue, while PayPal CEO Dan Schulman told Fortune back in April that his business had seen a “tremendous surge” during the pandemic.
Changes in consumer habits during the pandemic accelerates a trend that had already been in place for years: big data is now mission-critical to more and more businesses, not just the tech giants. From the machine learning algorithms that triage posts in Facebook newsfeeds to geo-targeted ads from local businesses, most B2B and B2C software applications rely on data to personalize the user experience.
Businesses, too, recognize the return on investment from grounding their business decisions in data. While many businesses eliminated non-essential spending in response to the pandemic, companies continued to invest in B2B software applications that give them more insight into their data such as project management tools, business intelligence, and marketing automation, with 27% reporting that spending had increased in 2020. While some of these software applications purport to be turnkey solutions, companies still hire data scientists to build custom data pipelines, create and maintain databases and own the overall data management strategy.
As organizations invest heavily in digital transformation—the integration of digital technologies into all areas of business—a plethora of data science sub-roles is cropping up, from data architect to business intelligence engineer, data engineer, database administrator, and niche machine learning specializations such as NLP engineer or computer vision specialist.
While data scientists are instrumental in helping corporations pad their bottom line, data science can also generate real social impact, from combating the opioid crisis or helping vulnerable families access public benefits. “There’s a big blue ocean of problems data scientists can tackle in fields that are most likely in line with something they’re passionate about,” says Sunischal Dev, a Springboard mentor and data scientist who works for Noodle, a company that uses AI to reduce industrial waste through supply chain management. In his spare time, he volunteers with Project Drawdown, running predictive models that measure the impact of the 100 most effective solutions to climate change—from wind and solar energy to regenerative farming practices—to help leaders make better policy decisions.
Get To Know Other Data Science Students
Karen Masterson
Data Analyst at Verizon Digital Media Services
Hastings Reeves
Business Intelligence Analyst at Velocity Global
Esme Gaisford
Senior Quantitative Data Analyst at Pandora
A talent shortage and a tight labor market
The talent shortage in data science isn’t a simple matter of not enough people training to become data scientists. In fact, there’s an “experience” gap that tends to be built into highly practical professions with a steep learning curve like software engineering, where education is a weak substitute for real-world experience.
“From what I’ve observed, technical skills alone will only get you halfway there,” says Dev, who has experience hiring for data science roles. “You really need to have a solid grasp of the domain or industry that you’re working in to be effective.”
Data from QuantHub indicates there was a shortage of 250,000 data science professionals in 2020. Some 35% of organizations surveyed said they anticipated having the most difficulty finding appropriate skillsets for data science roles, second only to cybersecurity.
While companies struggle to fill these roles, the demand for data-literate business professionals is expanding beyond traditional data science jobs. Companies seek candidates with analytics skills, such as data-minded digital marketers (hence the term “growth marketing”), HR professionals, account managers, and financial consultants: people who can query data for business insights, A/B test different approaches, track performance metrics, and show how they’re adding value to the bottom line.
PwC coined the term “analytics-enabled” jobs to describe this new breed of data-informed job roles.
C-level executives such as CEO and CIOs have always relied on key performance indicators to make decisions, but leadership teams are digging deeper into their data, relying on detailed dashboards, real-time business intelligence, and insights from data scientists. “These days what’s more effective is when data scientists are very integrated and embedded in the functional teams and they’re able to apply analysis side by side with stakeholders,” said Dev.

How is the industry responding?
The reasons behind a talent shortage are never cut and dry. In 2018, the number of data science job postings far outpaced the number of job seekers, according to Indeed. But the picture gets more complicated when factoring in employer expectations and the current education system. The gender gap in STEM fields applies equally to data science, according to a report by the Business Higher-Education Forum and PwC, where men outnumber women by three to one.
Universities have responded to the demand for data science talent by ramping up degree programs in data science, data analytics, and machine learning, particularly at the undergraduate level. Many undergrad computer science majors now study machine learning as part of their course, and universities have launched 303 new data science and analytics degrees and credentials since 2010. According to Data Science Programs, there are over 830 data science programs offered by over 500 universities, with the Master of Data Science being the most popular.
But this onslaught of education programming is not enough. Just 23% of educators say their graduates will have data science and analytics skills, and many of these programs have not been around long enough for employers to get a clear view of the viability of the job candidates they produce.
What’s more, many data scientists are self-taught and rely on MOOCs and other online courses to learn data science, which further fragments the job market and the types of qualifications employers find palatable. Over four million people have enrolled in the Data Science Specialization offered by Johns Hopkins University through MOOC provider Coursera. Some employers are willing to accept credentials from short courses; others worry candidates are missing fundamental analytics skills. Consequently, job seekers have trouble framing their skill set in a way that is favorable to recruiters.
However, the problem also lies with employers, who tend to demand very specific technical experience such as Hadoop or R instead of emphasizing more widely applicable skills like data visualization and teamwork. Since data science is often a decision-making role, employers tend to look for candidates with 5-10 years of experience, with less demand for junior positions. “There is a lack of readiness to invest in developing talent, employers expect people to know everything from the start,” said Maria Dyshel, co-founder and CEO of Tangible AI. “Everyone loses out.”
Companies like IBM, Cognizant, and Amazon are addressing the talent shortage by reskilling and upskilling their existing employees in analytics skills and artificial intelligence. This could be as simple as encouraging employees to take MOOCs or attend conferences to creating an in-house education curriculum or enlisting a third-party education provider. In some cases, employees in non-data-related roles are also encouraged to participate in data science training such as data visualization, AI, and information strategy.
One Shanghai-based company, Transwarp, an AI platform, launched its own university to provide data science training and certification programs for people looking to upgrade their skills. “I think it would be great if we teach more data literacy to everyone,” said Dev. “Kind of like how in the past couple of decades we’ve seen high schools are starting to teach basic Excel and Microsoft Office skills.”
Data science is a broad field that can’t be taught over the course of a few afternoon seminars. But what organizations can do is offer training to high-performing employees or those whose core skills are already tangentially related to data science, such as BI analysts or software engineers.
“I think that’s where the industry’s headed: it’s not about having a million proficient data scientists that can come up with the entire ETA from model creation to implementation,” said Colley. “It’s more about having software engineers that understand enough to implement these autoML techniques.”
But employer retraining programs are expensive and limited in scope and don’t help address the wider talent shortage. This is why online learning and bootcamps will play a crucial role in preparing the future workforce for the challenges technology will bring.
“General university curriculums should include some sort of data literacy,” said Dev. “Not necessarily coding but just being able to understand how data influences the decisions we make these days.”
Since you’re here…
Curious about a career in data science? Experiment with our free data science learning path, or join our Data Science Bootcamp, where you’ll only pay tuition after getting a job in the field. We’re confident because our courses work – check out our student success stories to get inspired.