These Machine Learning Startups Are Ushering in a Data Revolution
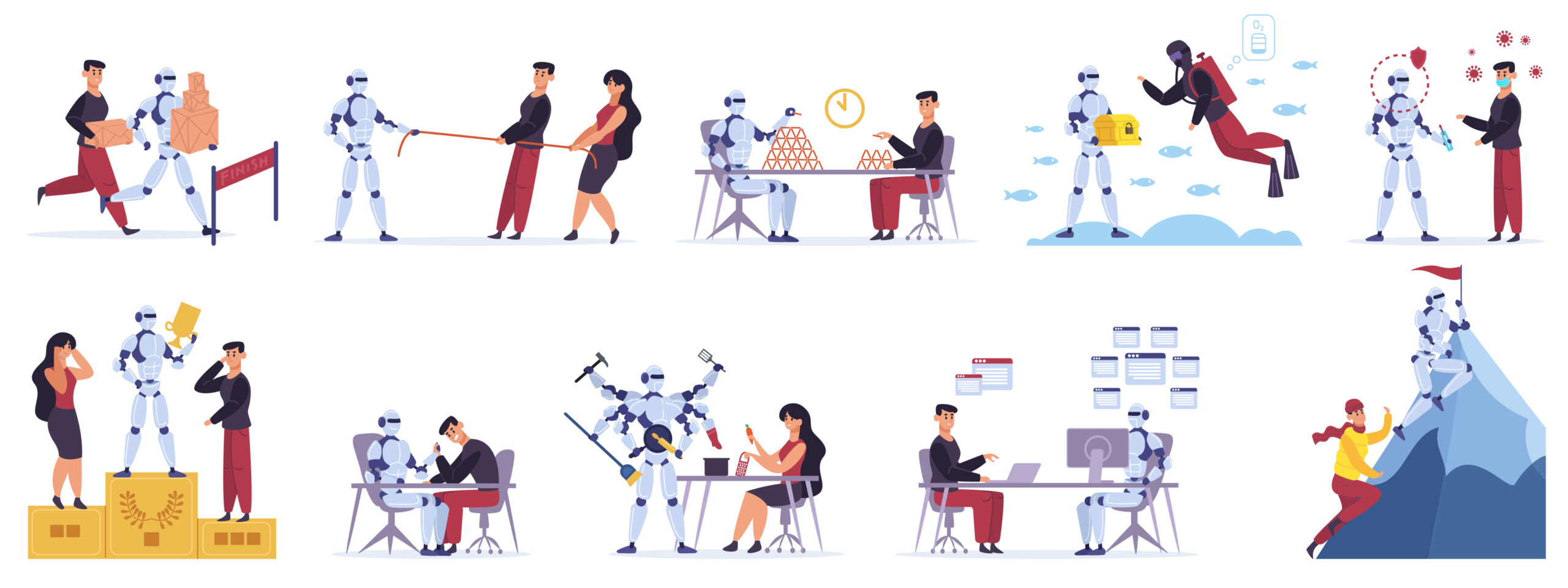
Plenty of organizations use big data to enhance their operations. Banks deploy data mining techniques to detect fraud or predict the likelihood a borrower will default on a loan. E-commerce companies apply quantitative and probabilistic methods to head off cybersecurity threats while mining massive amounts of customer data to build recommendation engines. Targeted marketing campaigns aimed at providing personalized customer experience.
But as the use cases for data science grow more sophisticated, some innovative startups are now relying on artificial intelligence and machine learning for their core product offering or business model—and in doing so, achieving things that wouldn’t be possible without data. Over 12,000 startups listed on Crunchbase rely on machine learning for their main and ancillary products and services. According to data from the National Venture Capital Association, AI-related companies in the U.S. raised $6.9 billion in Q1 of 2020.
Here are four ways that data-driven startups have been shaking things up.
Revolutionizing content marketing
Automation tools for writing have grown increasingly mainstream: Grammarly’s web browser extension flags poor sentence structure and redundant words in emails and documents, while the Google Workspace (formerly G Suite) has a text predictor that finishes your sentences for you. But what about an AI tool that can generate an entire blog post, churn out hundreds of product descriptions from a spreadsheet of product specs, or even write a sports news article based on game data?
Natural language processing is a type of machine learning that enables computers to understand human language in the form of text or audio. But with natural language generation (NLG), machines can write and generate their own speech.
NLG is already widely used for prosaic content generation, such as translating raw data into actionable insights for business intelligence dashboards or sending automated updates to clients regarding their financial portfolio. Beyond constructing simple sentences with “[insert here]” placeholders for the data, automated writing tools can generate a blog post from just a headline and even write Shakespearian sonnets.
This is done using pre-defined templates known as a narrative design. Social media posts, product descriptions, and poetry each have a different narrative design. Machine learning algorithms are trained using huge datasets to learn a narrative design—the typical structure, cadence, and word choices associated with a certain type of content. To generate new content, structured data is fed into the software and processed through the “conditional logic” that’s part of the narrative design.
“My goal is to allow content creators to just focus on creative ideas and leave the nitty-gritty details to the machines,” says Przemek Chojecki, CEO of Contentyze, an AI-powered text editor and content generation tool. A prolific writer who has published five books and authored over 300 articles on Medium, Chojecki says the tool is designed not to supplant content creators but “superpower them with new capabilities”—similar to the way auto ML and data robots help data scientists work more efficiently.
Natural language processing focuses on the interaction between data science and linguistics, working with unstructured data generated from conversations, speeches, social media posts, and so on. This type of data doesn’t fit neatly into the traditional rows and columns of a relational database, making NLP and NLG an exciting challenge for data scientists who are interested in working with language instead of just numbers.
“Natural language processing has changed a lot in recent years thanks to the introduction of transformers,” says Chojecki, referring to a deep learning model used for tasks like translation and text summarization. “Any data scientist out there interested in working with text data should learn NLP methods.”
Empowering small businesses to compete with corporations
What advantage does a large corporation have over a mom-and-pop store, aside from access to capital? Data. Springboard UX design alum Brenden Martin founded his own business, Joe Coffee, to help local coffee shops compete with national chains like Starbucks. Coffee shops sign up to the platform, install the app on a tablet or other device, and start receiving orders from customers in the local area. Meanwhile, customers receive recommendations for nearby coffee shops based on their GPS location.
More importantly, the business aggregates transaction data to provide customers with personalized offers through SMS, email, and push notifications—for which the average coffee shop owner has neither the time nor resources. Data insights help business owners determine things like best selling items or which items are typically ordered together, a crucial marketing function given that most coffee shops survive on repeat purchases. “Our job is to level the playing field with the national chains and be that tech and marketing hub so that business owners can concentrate on making great coffee and creating amazing relationships with their customers,” said Martin.
Get To Know Other Data Science Students
Meghan Thomason
Data Scientist at Spin
Jonathan King
Sr. Healthcare Analyst at IBM
Bryan Dickinson
Senior Marketing Analyst at REI
Using voice technology to overcome accessibility issues
When Matt Wiethoff, a software engineer, developed a repetitive strain injury in his wrists from years spent sitting at a computer for eight hours a day, he turned to dictation apps so he could continue coding while wearing a splint. But dictating code word for word is a painstaking process, and he was “far from fully productive.”
Wiethoff realized he’d discovered a gap in the market; at the time, there was no such thing as a speech-to-text translator designed specifically for web developers. In 2018, he co-founded Serenade, an AI-powered tool that allows coders to write code using their voice. Instead of relying on just dictation, the tool is trained to recognize natural language so you can describe manipulations to existing code like “delete class” or “add parameter URL.” The software is trained in common programming constructs, variable names, and other words used to write code.
“We wanted to create a product that was really easy to use, to the point where you could speak naturally, as you would in a conversation, and code would be written for you,” Wiethoff wrote in a blog post.
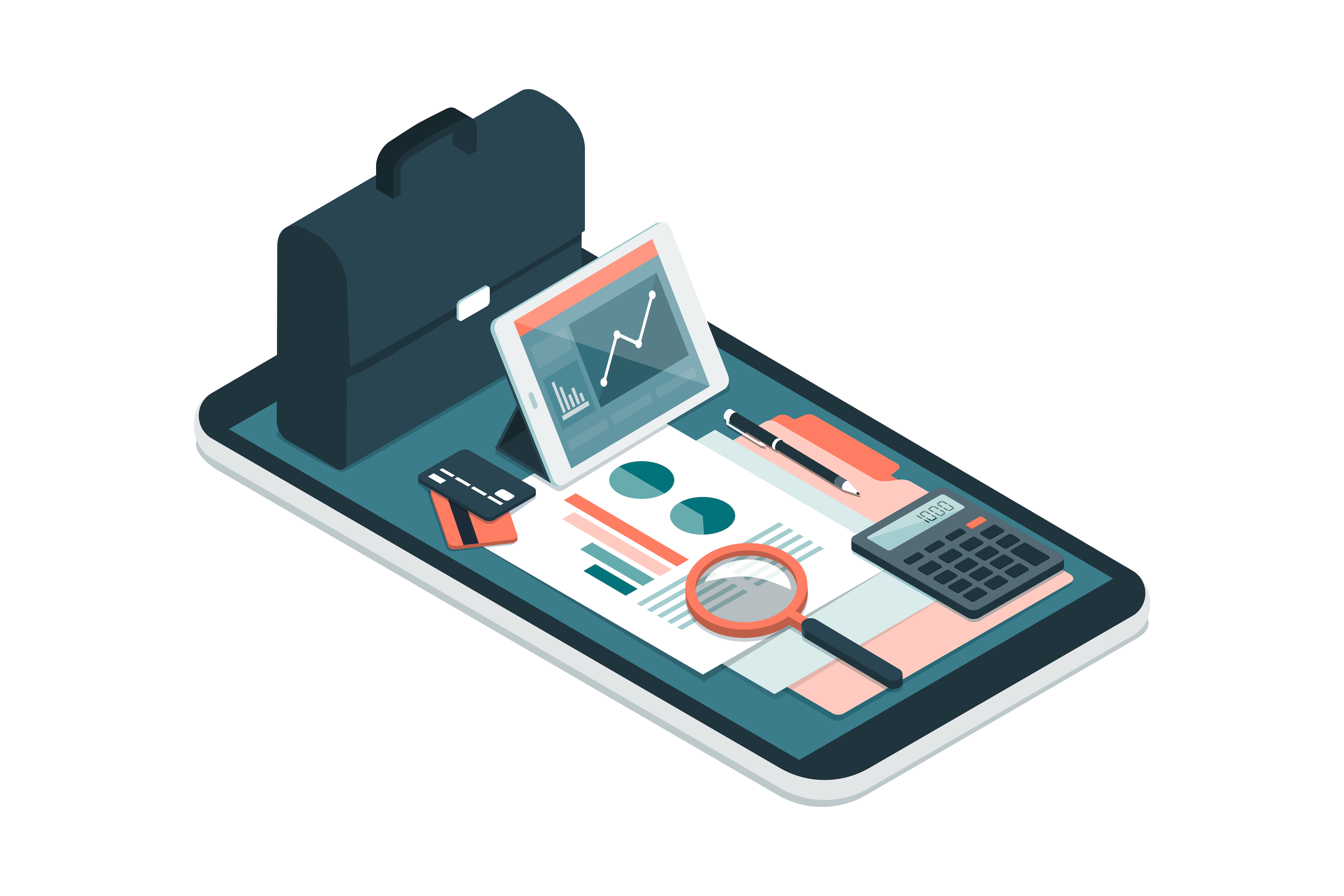
Using data on rent payments to build credit
When Wemimo Abbey arrived in the U.S. from Nigeria, he needed to borrow money to pay for his first year of college. With no credit history to show for, he ended up taking out a loan with an interest rate of over 400%. Today, he runs a fintech company, Esusu, whose mission is to use data to disrupt the vicious cycles endured by the low-income community, where bad credit leads to predatory lending, making it more difficult to build wealth or own a home.
Esusu’s mobile app comes with a rent reporting feature designed to help renters build credit by turning their rent payments into a credit-building activity.
“We work with large multifamily [property] owners and operators to capture rental payment data and report it to the credit bureaus to help tenants build and establish their credit scores today,” said Samir Goel, who co-founded the company with Abbey in 2018. “A loan provider can look at someone and be like, wow, this person has rented their home for 20 years; I bet they’re a really good bet for this mortgage product.”
A raft of startups has sprung up in recent years designed to help people make sense of their financial data to help with budgeting, saving, and investing outside of the traditional banking system. From chatbots like Cleo that play the role of a financial advisor to apps like Varo Money, which provides an automated savings feature and a dashboard that helps users track how much they spend and save, putting data in the user’s hands empowers people and organizations to make better decisions.
Since you’re here…
Thinking about a career in data science? Enroll in our Data Science Bootcamp, and we’ll get you hired in 6 months. If you’re just getting started, take a peek at our foundational Data Science Course, and don’t forget to peep our student reviews. The data’s on our side.