8 Python Libraries for Data Science Worth Knowing in 2023
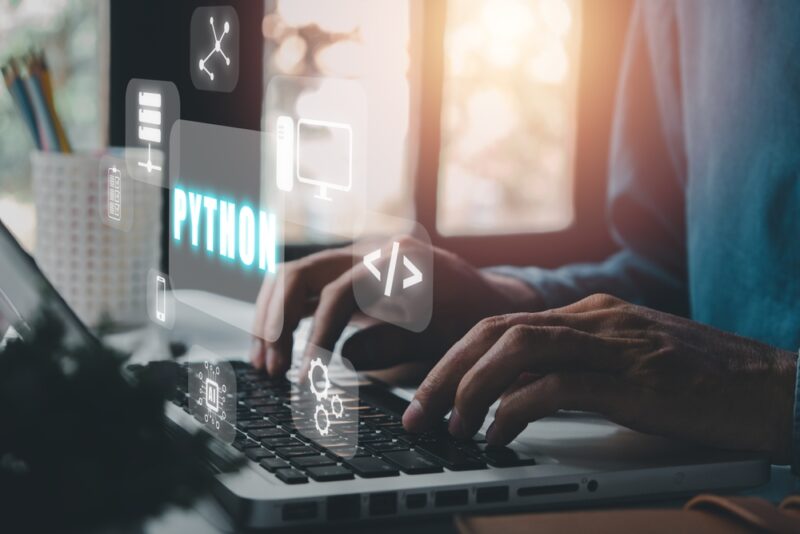
In this article
Collections of prewritten code, more commonly known as programming libraries, are indispensable tools for virtually all programmers. But for data scientists working with Python, the value of libraries is on another level. Whether you’re doing data transformation, analysis, machine learning, or visualization, these libraries are essential.
Of course, with so many out there, it can be a little overwhelming to choose the right one. That’s why we’ve put together this guide. Below, we’ll tell you all about the 8 best python libraries for data science, and what makes them so great.
What Is a Python Library?
Python libraries are collections of modules that programmers can use to perform tasks while writing fewer lines of code. Python is famous for having a massive amount of libraries, totaling over 137,000, which are used extensively in many fields. Most of the most popular Python libraries, however, are tied to the field of data science.
8 Popular Python Libraries for Data Science
Here are the 8 best Python libraries for data science.
NumPy
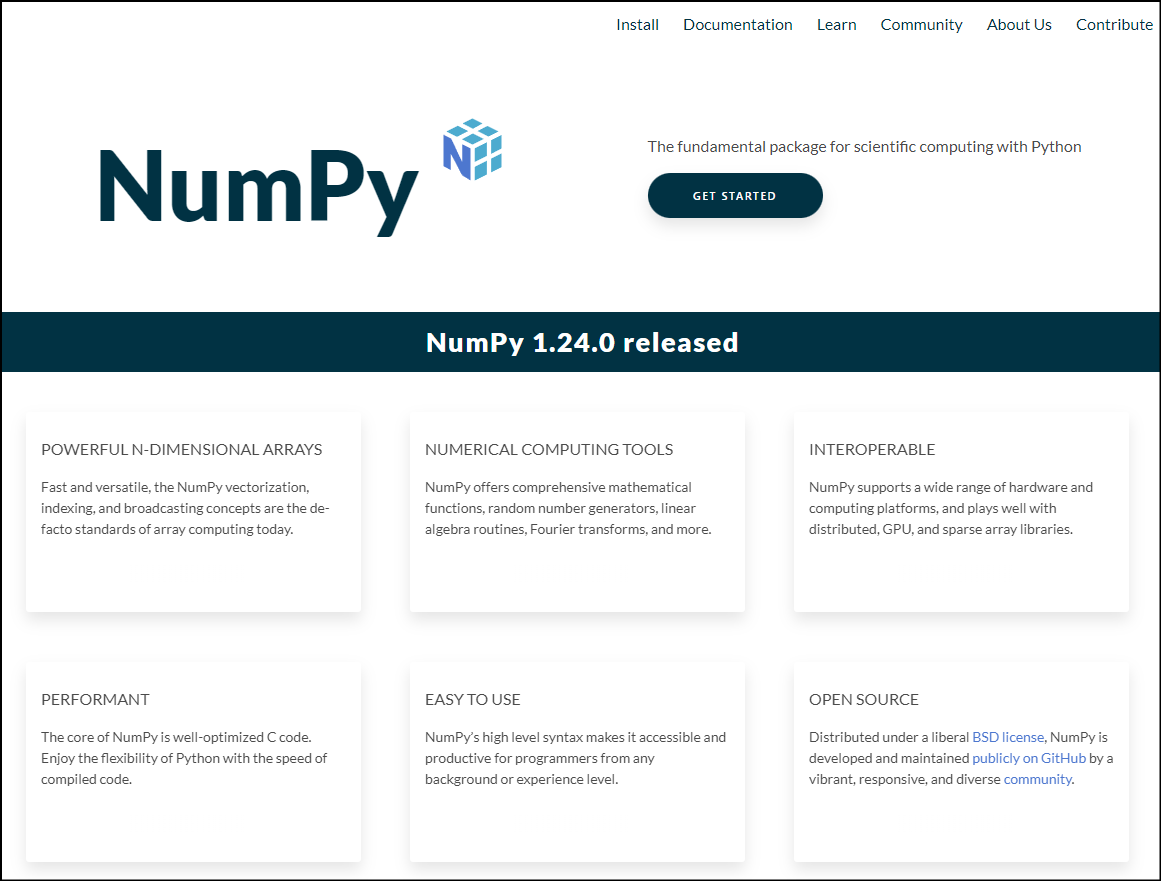
NumPy (Numerical Python) is an open-source package that allows numerical computing in Python, including mathematical functions, random number generators, and linear algebra routines. The library itself is written with C code, which runs much faster than Python. This allows users to access the speed of compiled code, while still getting to use the simple and user-friendly Python syntax.
Libraries often build off other libraries, and the computational power NumPy provides is at the core of many other Python data science libraries, including pandas, scikit-learn, and SciPy. It’s also an essential component in visualization libraries like Matplotlib and seaborn and allows users to visualize bigger datasets than Python could handle alone.
Highlights
Some of the many tasks NumPy can achieve include:
- Statistical computing
- Signal processing
- Mathematical analysis
- Image processing
- Graphs and networks
- Bayesian inference
- Multidimensional arrays
pandas
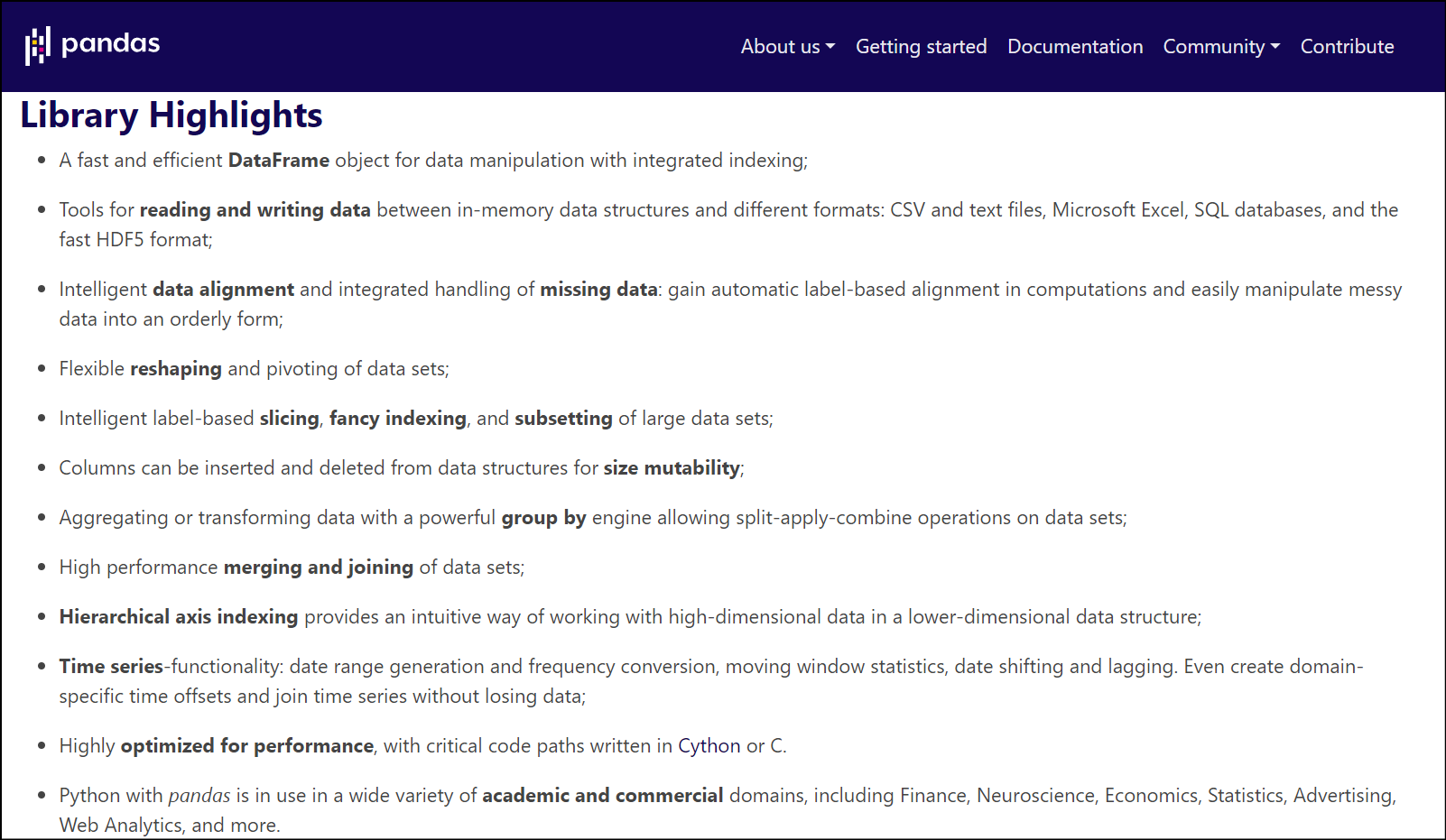
pandas is an open-source and NumFOCUS-sponsored project that began in 2008. It aims to provide programmers with the building blocks needed to complete practical, real-world data analysis in Python. Like NumPy, pandas is written with C code, so users can experience powerful and fast results while writing flexible Python code.
The pandas library is generally used to extract, transform, and load data at the beginning of the data science process. It possesses tools for reading and writing data between various structures and formats such as text files, Excel files, and SQL databases.
If you’re interested in learning more about pandas, then you should definitely check out Springboard’s Data Science Bootcamp, which teaches students how to pandas to wrangle and clean data as part of its 500+ hour curriculum.
Highlights
- Hierarchical axis indexing
- Merging and joining of data sets
- Aggregating and transforming data
- Label-based slicing, fancy indexing, and subsetting
- Intelligent data alignment
- Flexible data structures
- Fast and efficient DataFrame
Matplotlib
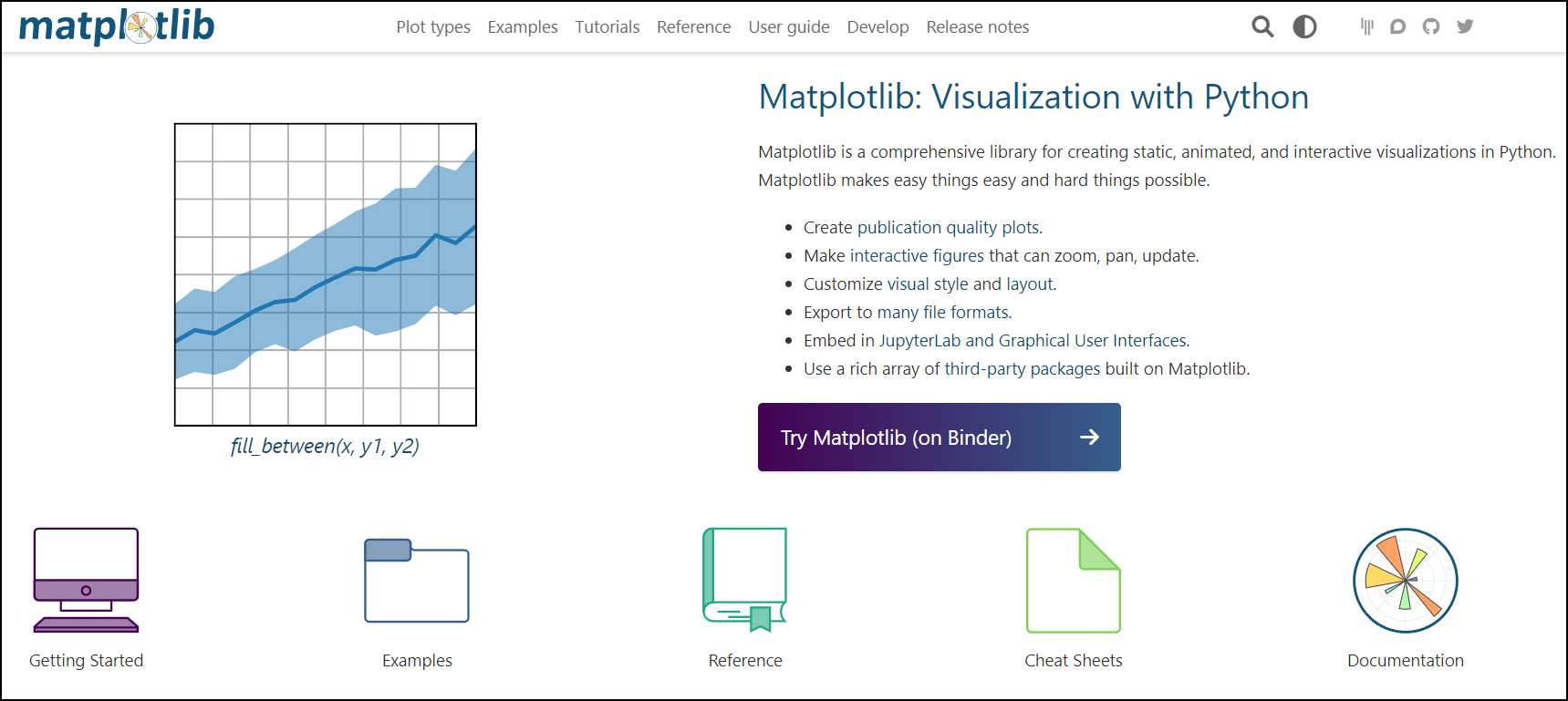
Matplotlib is a library for creating data visualizations in Python. It can generate static, animated, and interactive plots of high quality. The library has a high-level interface to increase accessibility for users of all levels and abilities. A wide range of different plot types are available, including but not limited to:
- Scatter plots
- Bar charts
- Pie charts
- Box plots
- Error charts
- Stem plots
- Contour plots
- Joint plots
- Stackplots
- Streamplots
Visualization libraries are used at the end of the data science process to present the data and derived insights in a clear and digestible format. These plots, graphs, and two-dimensional diagrams are shown to decision-makers during a data scientist’s presentation to help viewers understand the data and make decisions based on it. Matplotlib can also embed plots into applications on desktops and mobile devices using an object-oriented API.
Highlights
- Create high-quality plots
- Make Interactive figures that can zoom, pan, and update
- Utilize lots of third-party packages
- Customize visual style and layout
- Create computational graphs
- Export to many different file formats
SciPy
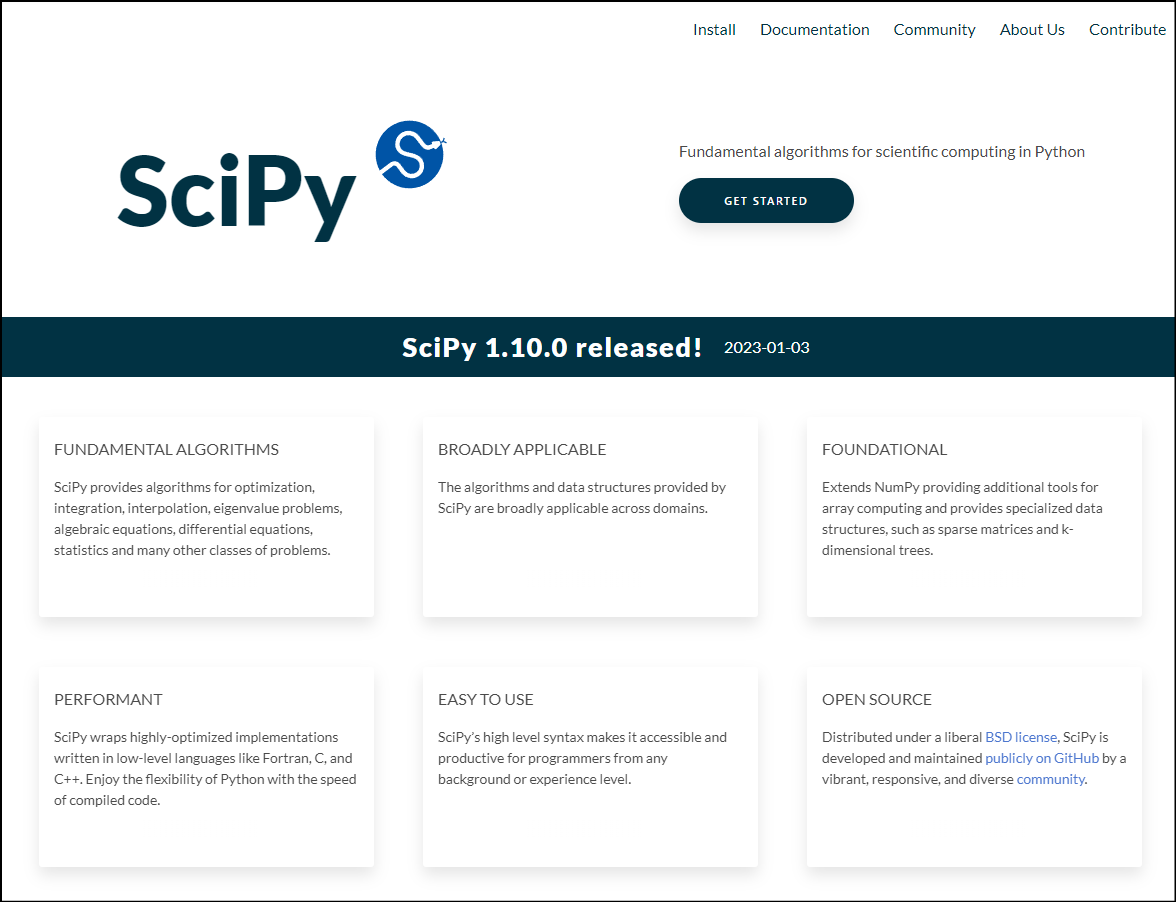
SciPy (Scientific Python) is a sister project of NumPy that focuses on scientific computing with Python. It builds on NumPy, providing additional manipulation tools for solving mathematical, scientific, engineering, and technical problems. It also works with array computing, algorithms, and high-level data structures such as sparse matrices and k-dimensional trees.
The library is written with multiple low-level programming languages like Fortran, C, and C++ to combine the speed of compiled code with the flexibility of Python, just like NumPy. With high-level syntax, SciPy is accessible and usable for programmers of many different levels and backgrounds.
Highlights
SciPy includes algorithms for a variety of uses such as:
- Optimization
- Integration
- Interpolation
- High-level commands
- Eigenvalue problems
- Advanced array operations
- Algebraic equations
- Differential equations
- Statistics
Seaborn
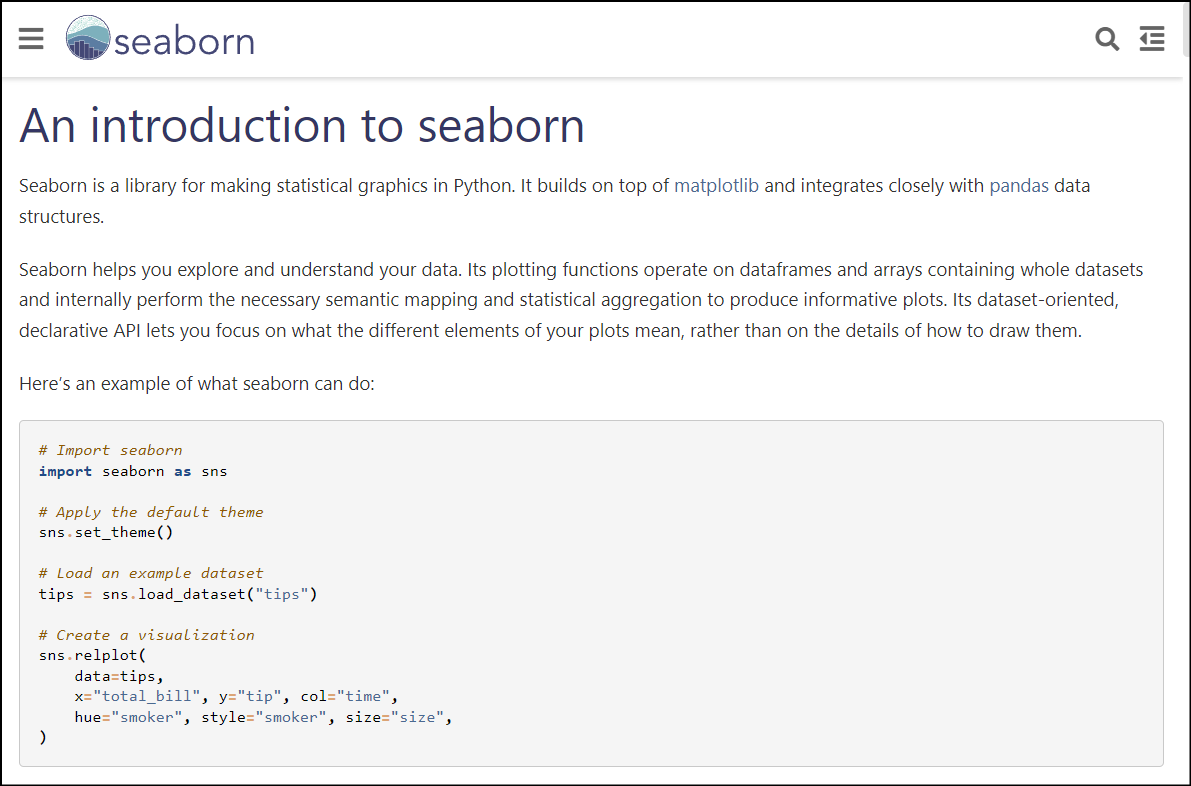
Built on top of Matplotlib and drawing on pandas data structures, the Seaborn plotting library is used for generating informative statistical graphics in Python. It focuses on simplifying complex visualizations and adding extra aesthetic customizations for even more professional-looking plots.
Seaborn comes with a number of examples that dataset programmers can use to start learning how to visualize data, so it’s easy for newcomers to get to know the library.
Highlights
Like Matplotlib, Seaborn makes a variety of different plot types available to its users, including:
- Scatter plots
- Histogram plots
- Bar charts
- Box plots
- Violin diagrams
- Error charts
- Facet grids with distplot
- Pair plots
- Bubble charts
- Pie charts
- Cluster maps
- Heatmaps
PyTorch
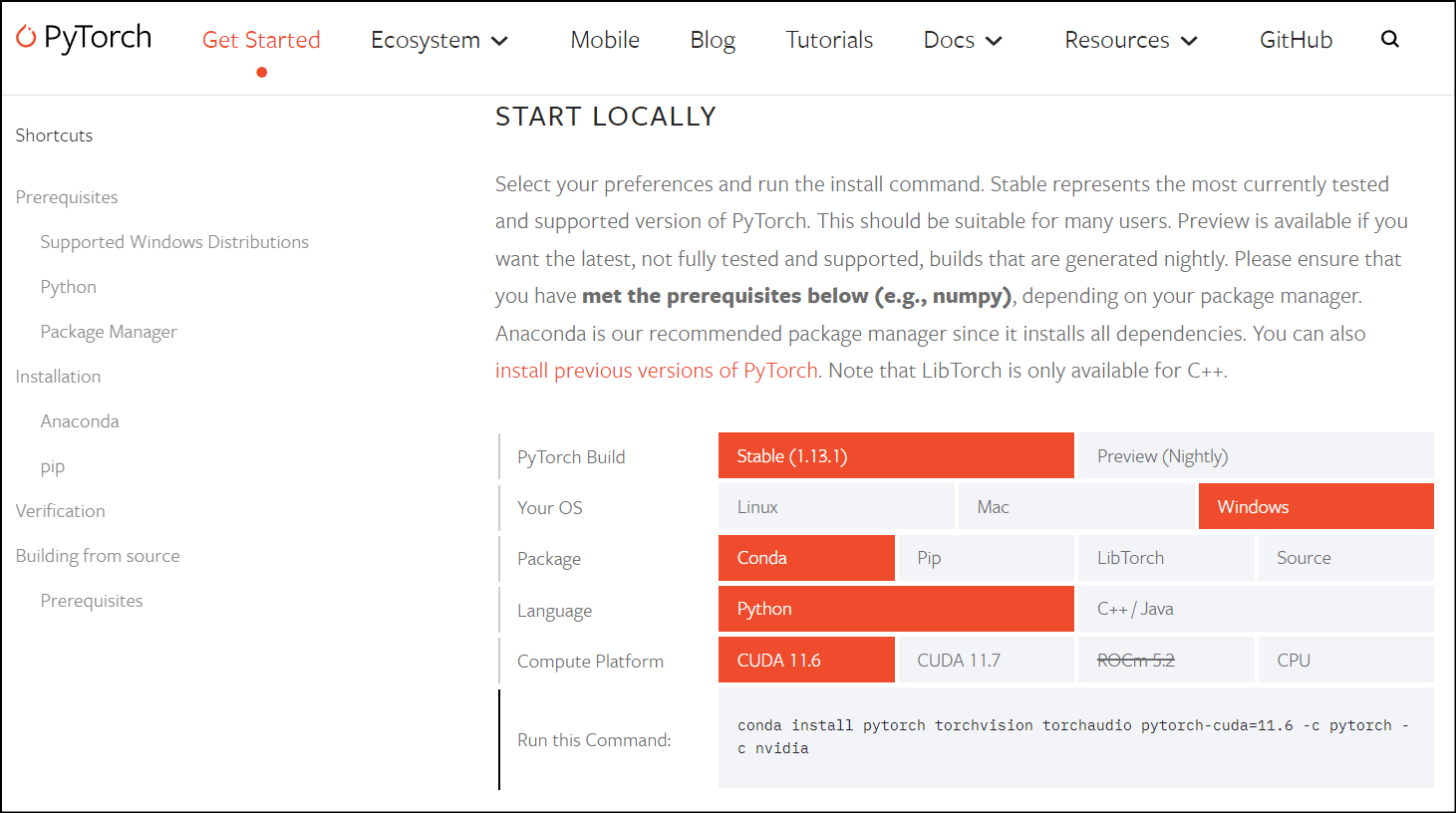
PyTorch is an open-source machine learning framework and deep learning library used by big names such as Amazon, Salesforce, and Stanford University. The project is part of the Linux Foundation and enables fast and flexible production of machine learning models.
The library can be used either with the default Python frontend or a C++ frontend that allows the users to interact with the library by writing C++ code.
Highlights
- Easy-to-use TorchScript
- TorchServe for easy deployment
- Distributed training
- Experimental mobile feature
- Tensor computations with GPU acceleration
- Robust ecosystem and active community
- Native ONNX support
- C++ frontend
- Natural language processing
- Cloud support
TensorFlow
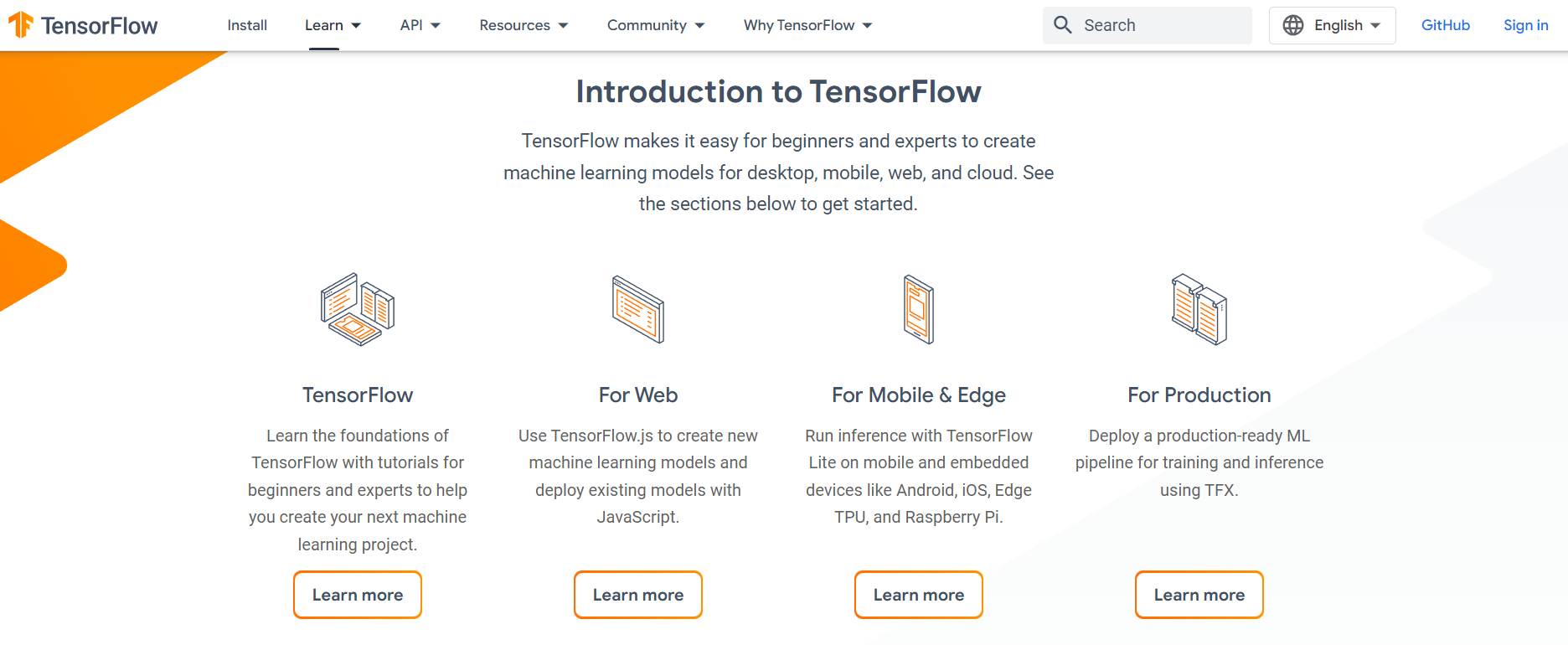
TensorFlow is a popular open-source library for machine learning that helps users create production-grade deep learning models more quickly and easily. The library provides tutorials, examples, and various other resources to speed up build times and create scalable deep-learning models. Users can search for pre-trained models or build and train their own based on what they need.
Users can join the active community by contributing to forums and user groups, attending machine learning tech talks, joining a special interest group, or becoming a contributor. There’s also a collection of add-on libraries and models for users to draw on, including Regged Tensors, TensorFlow Probability, Tensor2Tensor, and BERT.
Highlights
- Easy model building
- Robust ML production
- Powerful Experimentation
- Statistical models
- Pre-trained models
- ML solutions for every skill level
- Implement MLOps
scikit-learn
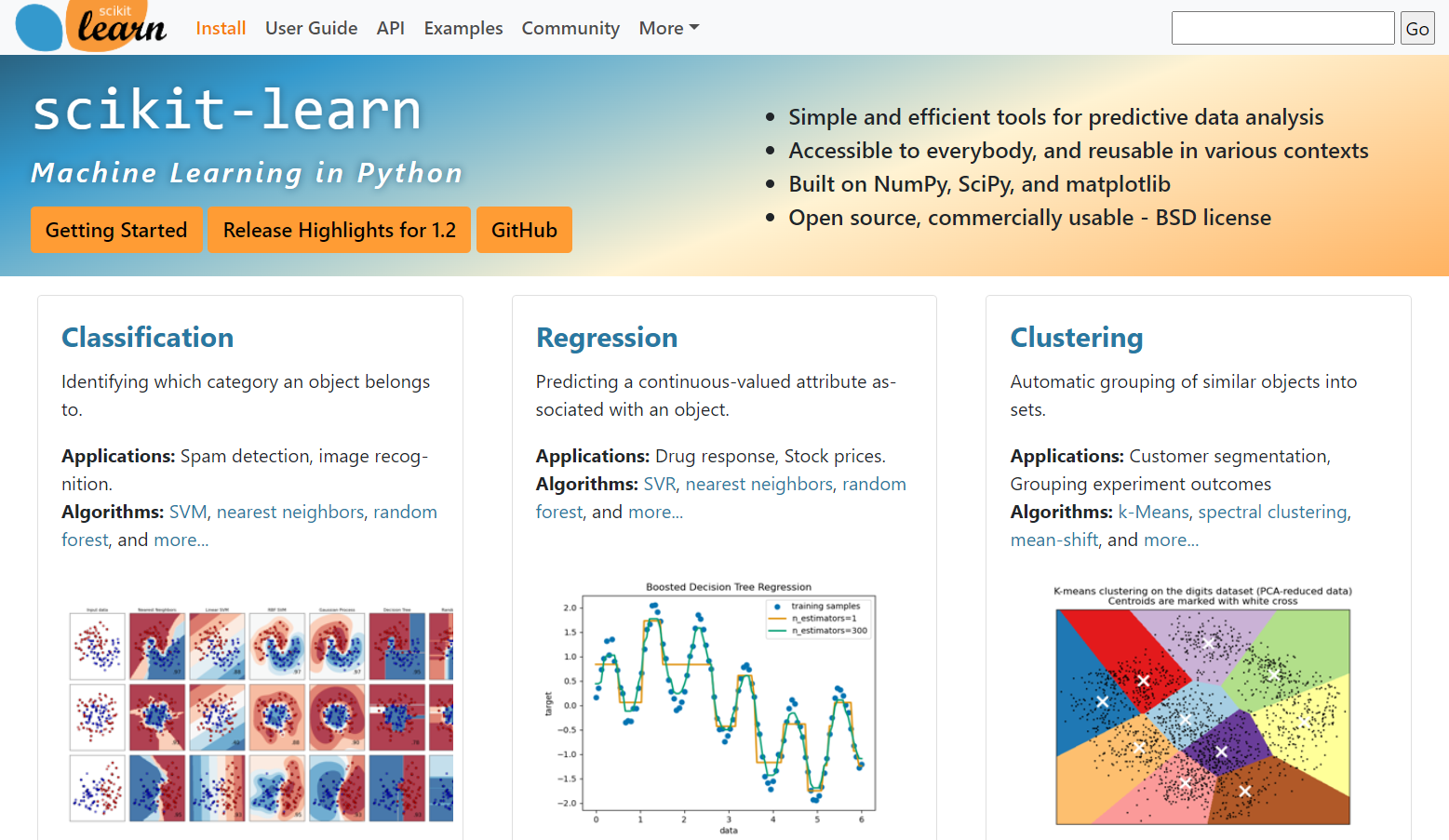
scikit-learn is another machine-learning library that provides simple and efficient tools for predictive data analysis. Unlike a lot of the libraries listed, the fundamental package is largely written in Python and it’s built on NumPy, SciPy, and Matplotlib.
It was originally started as a Google Summer of Code project in 2007, with its first public release in 2010. It’s completely open source and funded by both its community and external organizations like Microsoft.
The library focuses on modeling data, using a number of features such as supervised learning algorithms, unsupervised learning algorithms, cross-validation, and ensemble methods.
Highlights
- Classification using Python
- Regression, used for datasets like stock prices
- Clustering for customer segmentation and grouping experiment outcomes
- Dimensionality reduction for visualization and increased efficiency
- Model selection for improved accuracy
- Preprocessing for transforming input data
Get To Know Other Data Science Students
Diana Xie
Machine Learning Engineer at IQVIA
Jonas Cuadrado
Senior Data Scientist at Feedzai
Aaron Pujanandez
Dir. Of Data Science And Analytics at Deep Labs
Popular Python Libraries for Different Applications
There are multiple stages in the data science process, and different libraries are used to help with each stage. Usually, the process looks something like this:
- Extract, transform, load (ETL)
- Data exploration
- Data evaluation
- Data modeling
- Data presentation (or visualization)
What Are the Top Python Libraries for Data Visualization?
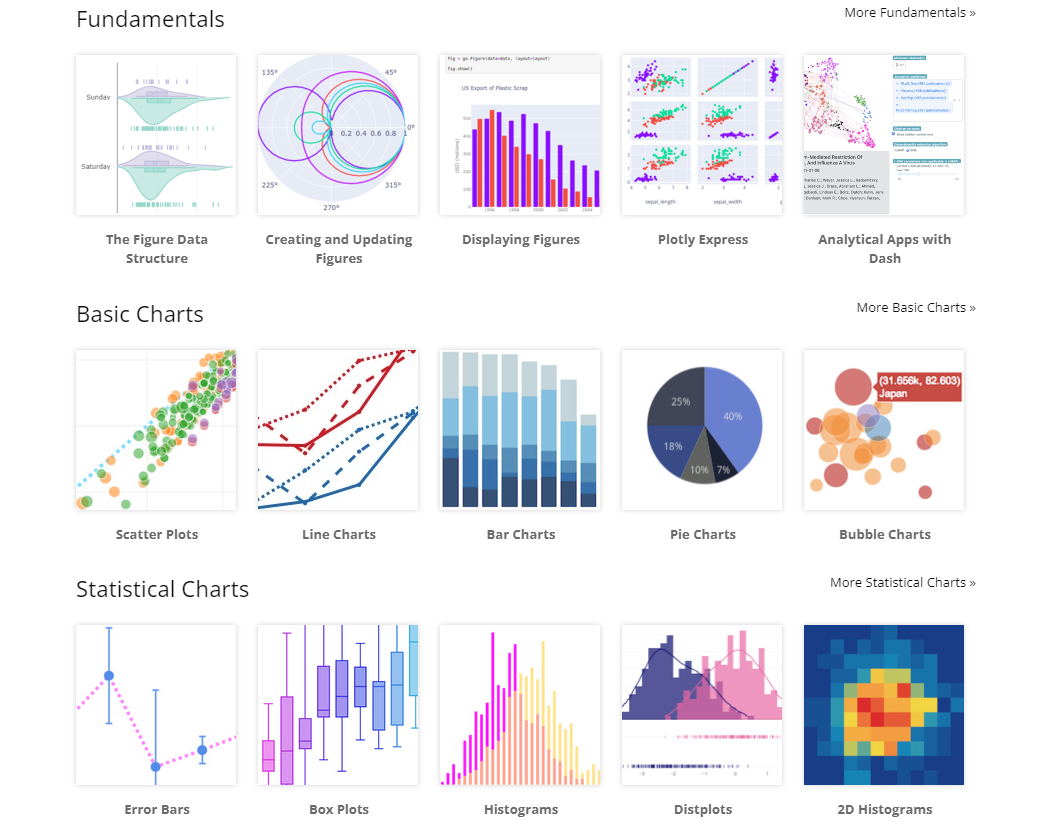
Matplotlib is usually seen as the top library for data visualization, and many libraries catering to more specific uses are built on top of Matplotlib. Other popular visualization libraries and low-code libraries include:
What Are the Top Python Libraries for Big Data?
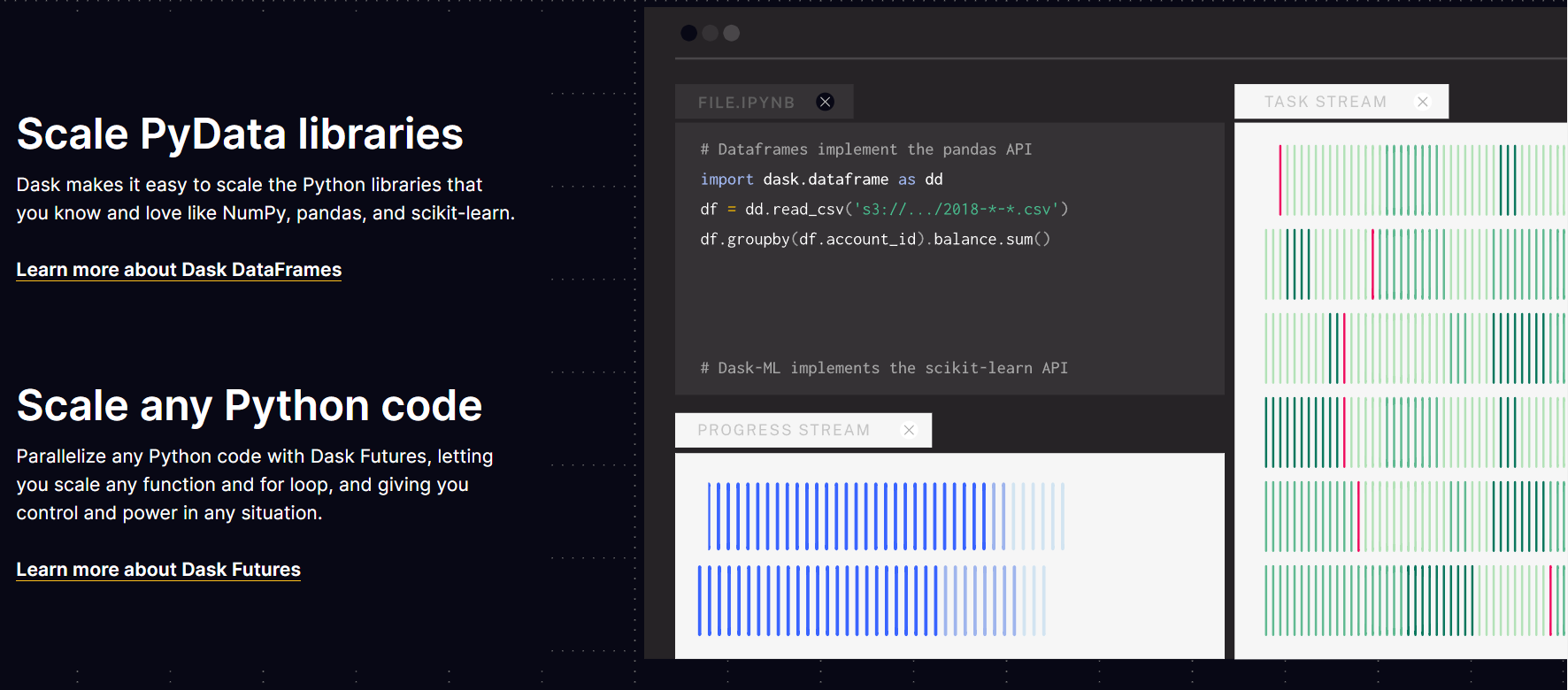
Working with particularly large datasets often requires specific libraries that can deal with the high volumes. Dask and Ray are two popular libraries that specialize in scaling complex workloads for big data. Other options include:
- TensorFlow
- pandas
- NumPy
- SciPy
What Are the Top Python Libraries for Data Engineering?
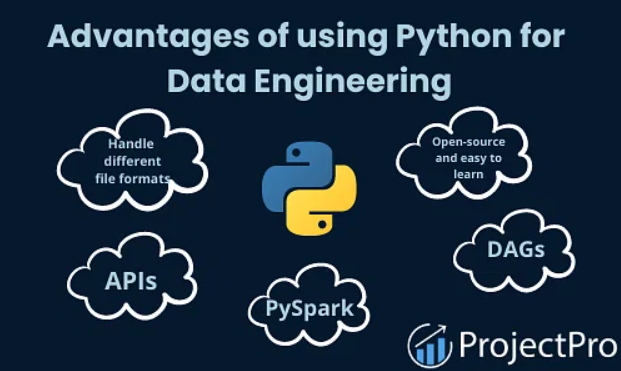
A data engineering project will likely use a range of libraries for different stages of the process. Here are some popular libraries often used for data engineering:
- Pandas
- Dask
- Tensorflow
- PyTorch
FAQs About Python Libraries for Data Science
Here are some frequently asked questions about Python libraries for data science.
What Should I Learn First: Pandas or NumPy?
Learning the basics of NumPy is a great place to start because the majority of other data science Python libraries use NumPy for their numerical computing. By understanding this foundation, you’ll also be able to understand more about what’s going on in the subsequent libraries you learn.
What Are the Best Python Libraries for Beginners?
Any of the most popular Python libraries—such as pandas, NumPy, SciPy, Matplotlib, PyTorch, and scikit-learn—are perfect for beginners, as they all focus on accessibility and ease of use. Each project aims to provide features for every level of programmer, to help them grow and be productive.
How Fast Can I Learn Python for Data Science?
Python has a high-level, simple syntax that is great for new learners and anyone new to programming. This means you can begin learning and start writing programs straight away, with the programs you write becoming more complex as you learn more and more. To master enough Python to take on a data science project, it would take somewhere between 6-8 months.
What Are Some Underrated Python Libraries for Data Science?
Some well-received but underrated Python libraries and packages include Emmett, Jam.py, Shogun, Blaze, and Altair. They focus on a range of data science tasks, including machine learning, dashboards, and web frameworks.
Since you’re here…
Thinking about a career in data science? Enroll in our Data Science Bootcamp, and we’ll get you hired in 6 months. If you’re just getting started, take a peek at our foundational Data Science Course, and don’t forget to peep our student reviews. The data’s on our side.